About
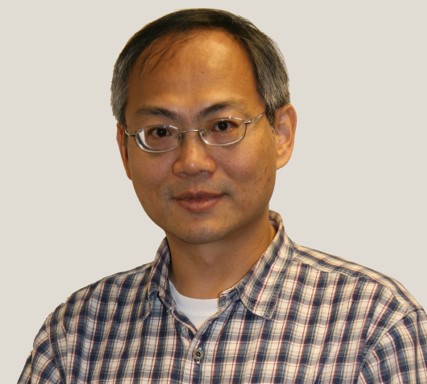
Yi-Kuo Yu, PhD
Senior Investigator, Computational Biology BranchDr. Yu’s lab investigates various biological problems at multiple levels of detail in order to gain quantitative understanding in biology. They develop solid foundation for quantitative understanding of biomolecular interactions; to better separate information from noise in massive biological data sets, they develop/employ computational approaches with sound statistical foundations. A major goal is to foster a solid connection between medical research and fundamental scientific research.
Contact Information
Building 38A, 8600 Rockville Pike, Room 6S610, MSC 3829 Bethesda, MD 20894
Tel: 301-435-5989
Research Interests
As a theoretical physicist working in biology, I have always been fascinated by the functional and organizational complexity achievable in a myriad of diverse organisms, all of which share a universal set of building blocks (water, ions, saccharides, fatty acids, amino acids, nucleotides and other small molecules). Despite vast amounts of effort invested, our current understanding of biology, ranging from a microscopic level to a more macroscopic level, is far from complete. For example, in terms of molecular interactions, phenomenological treatments at the intra- and inter-protein level have not yet fostered an effective theory capable of predicting how proteins fold and how a protein complex organizes itself. Namely, a proper coarse-graining procedure to bring out only the relevant degrees of freedom is lacking. This may be due to our insufficient understanding of biology in terms of relevant physics and chemistry, or it may be because the search for higher organizing principles is hindered by our inability to bring out information buried in noise and/or by conflicting interpretations of data. Developing methods to improve information extraction and establishing a firm foundation at the microscopic level are essential. Our approach to mitigate the issue of noise (or conflicting information) is to devise data analysis methods that can assign accurate statistical significance to signals identified; our take on building a firm foundation at the microscopic level is via development of calculation methods whose accuracy can be controlled. All our research efforts center about these two main emphases.