Speakers
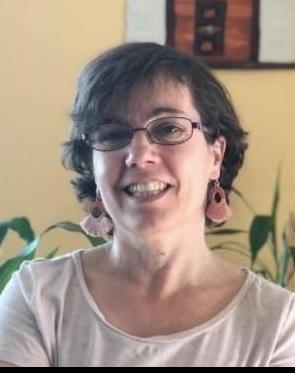
Cecilia Arighi
University of Delaware
Scalable literature integration and biocuration at the Protein Information Resource (PIR)
Abstract: The Protein Information Resource (PIR) is an integrated public bioinformatics
resource to support genomic,
proteomic and systems biology research and scientific studies. Many of the
projects at PIR, such as UniProt,
iPTMnet, Protein Ontology involve biocuration and/or integration of the
biomedical literature. In this
context, text mining has played an important role in assisting in the triaging
of publications, in the
extraction of relevant facts, and in presenting the publications in an organized
fashion. Reuse is key
and thus, we try to collaborate with text mining community to adopt existing
tools or customize/develop
new ones when needed. In UniProt, text mining is used to select the most recent
and relevant papers from
high impact journals, for triaging and for improving coverage and access to
literature and annotations in
protein entries. In iPTMnet, which integrates data about protein modifications
from a variety of databases
and text mining, the phosphorylation information extracted solely from text
mining source are presented to
curators to quickly validate and add a unique subset of substrate, sites, and
kinase information. Collecting
related facts across the literature and integrating results can be challenging.
We have developed the
iTextMine an automated workflow to integrate text mining tools from large-scale
text processing to offer
an effective way to query the literature and extract information on specific
bio-entity relations
(e.g., substrate-site-kinase; phosphorylation-dependent protein-protein
interactions, and miRNA-target).
APIs can be used to integrate iTextMine results into biocuration workflows or
literature services.
In this presentation, I will provide an overview of these projects and the way
text mining is being utilized
to drive scalability, and will reflect on lessons learned.
Bio: Dr. Cecilia Arighi has a B.S. degree in molecular biology, and a Ph.D. in biochemistry from the University of Buenos Aires, Argentina. She moved to the US to conduct a post-doctoral research in cell biology field at NICHD, National Institutes of Health. In 2005, Dr. Arighi joined the Protein Information Resource (PIR) as a biocurator and was appointed Research Assistant Professor of Biochemistry and Molecular & Cellular Biology at Georgetown University Medical Center. Still within PIR, she moved to University of Delaware (UD) in 2009, where she is currently a Research Associate Professor at the Center for Bioinformatics and Computational Biology. Dr. Arighi has extensive experience in the areas of database curation, community annotation, text mining for biocuration and ontologies. She is the lead of curation and text mining efforts at the Protein Information Resource. Dr. Arighi is a former Chair of the International Society for Biocuration. She is currently a member of the editorial board for the journal Database and the Europe PubMed Central Advisory Board. She has been an organizer and principal investigator of the BioCreative challenge since 2009. She serves as chair in the Library Faculty Senate Committee at University of Delaware. Dr. Arighi is currently involved in efforts to improve coverage and access to literature and annotations in UniProt entries via interaction with other databases, use of text mining and community crowdsourcing. In addition, she participates in the development and evaluation of text mining systems to assist in the retrieval of information about genes, proteins and miRNAs to assist data integration and biocuration at PIR.
https://bioinformatics.udel.edu/people/personnel/cecilia_arighi/
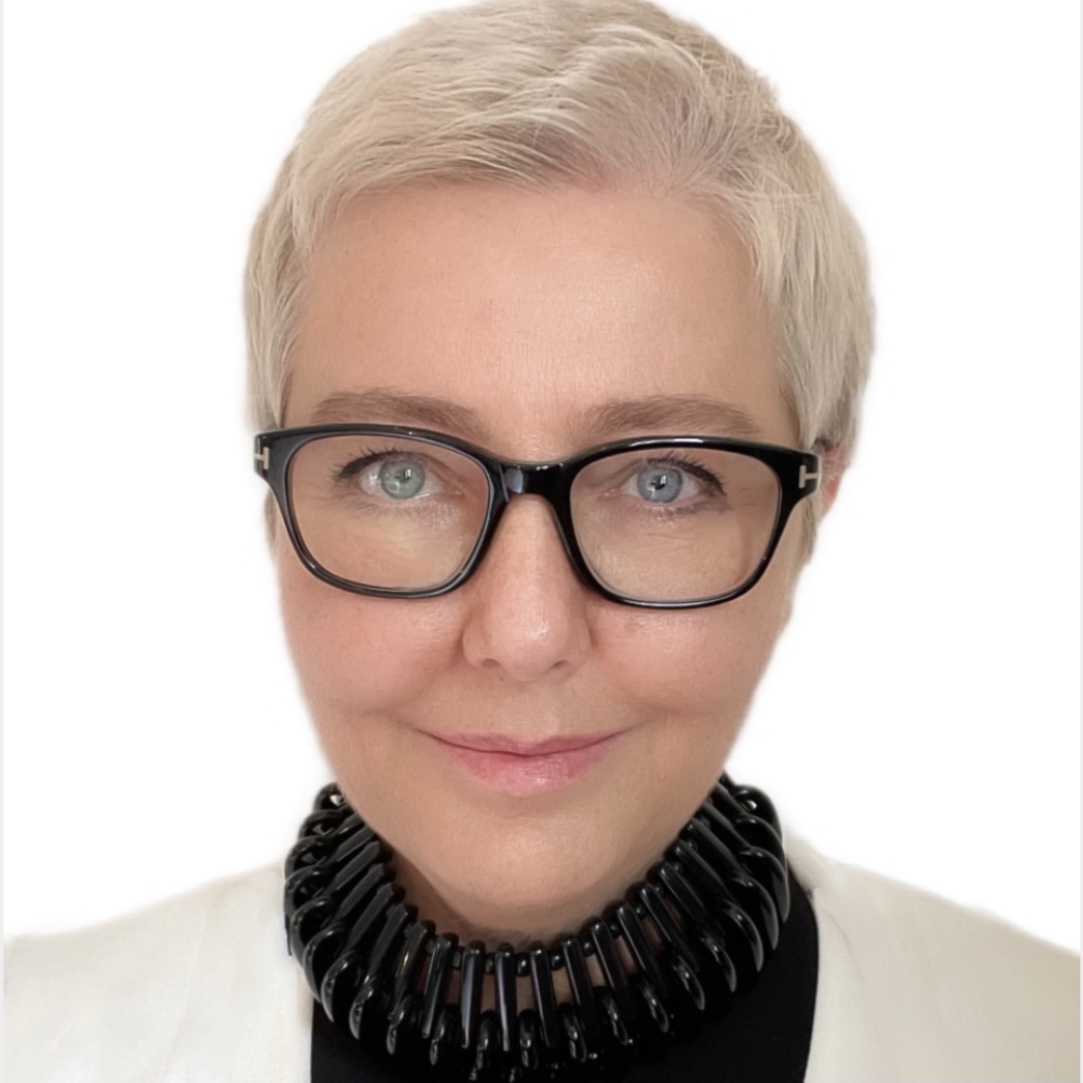
Susana Assunta-Sansone
University of Oxford
Bio: Susanna is a Full Professor in the Department of Engineering Science of the University of Oxford, and an Associate Director and Principal Investigator at the Oxford e-Research Centre. She is also a Consultant for Springer Nature, and Founding Honorary Academic Editor of the Scientific Data journal; and an ELIXIR Interoperability Platform Lead. Her Data Readiness Group (https://datareadiness.eng.ox.ac.uk) focuses on data sharing and reproducibility and the evolution of scholarly publishing, which drive science and discoveries. More specifically, they research and develop new ways to make digital research objects (including data, software, model and workflows) FAIR.
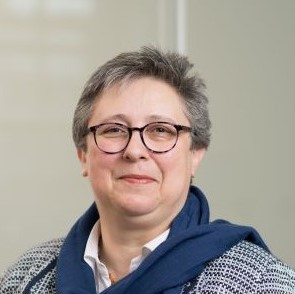
Theodora Bloom
The BMJ
Bio: Theodora Bloom is executive editor of The BMJ. She has a PhD in developmental cell biology from the University of Cambridge and worked as a postdoctoral fellow at Harvard Medical School, researching cell-cycle regulation. She moved into publishing as an editor on the biology team at Nature, and in 1992 joined the fledgling journal Current Biology. After a number of years helping to develop Current Biology and its siblings Structure and Chemistry & Biology, first for Current Science Group and then for Elsevier, Theo joined the beginnings of the open access movement. As the founding editor of Genome Biology she was instrumental in the birth of the commercial open access publisher BioMed Central, where she remained for several years, ultimately as editorial director for biology. After a spell as a freelance publishing consultant working with a variety of clients, she joined the non-profit open access publisher Public Library of Science (PLOS) in 2008, first as chief editor of PLOS Biology and later as biology editorial director with additional responsibility for PLOS Computational Biology and PLOS Genetics. She also took the lead for PLOS on issues around data access and availability, introducing PLOS’s pioneering data availability policy. At The BMJ her responsibilities include publishing, business, platform and operations, as well as ethical and policy matters and dealing with complaints. She is a Co-Founder of the medRxiv preprint server, a collaboration between BMJ, Cold Spring Harbor Laboratory and Yale University, and jointly coordinates open access and open research initiatives at BMJ.
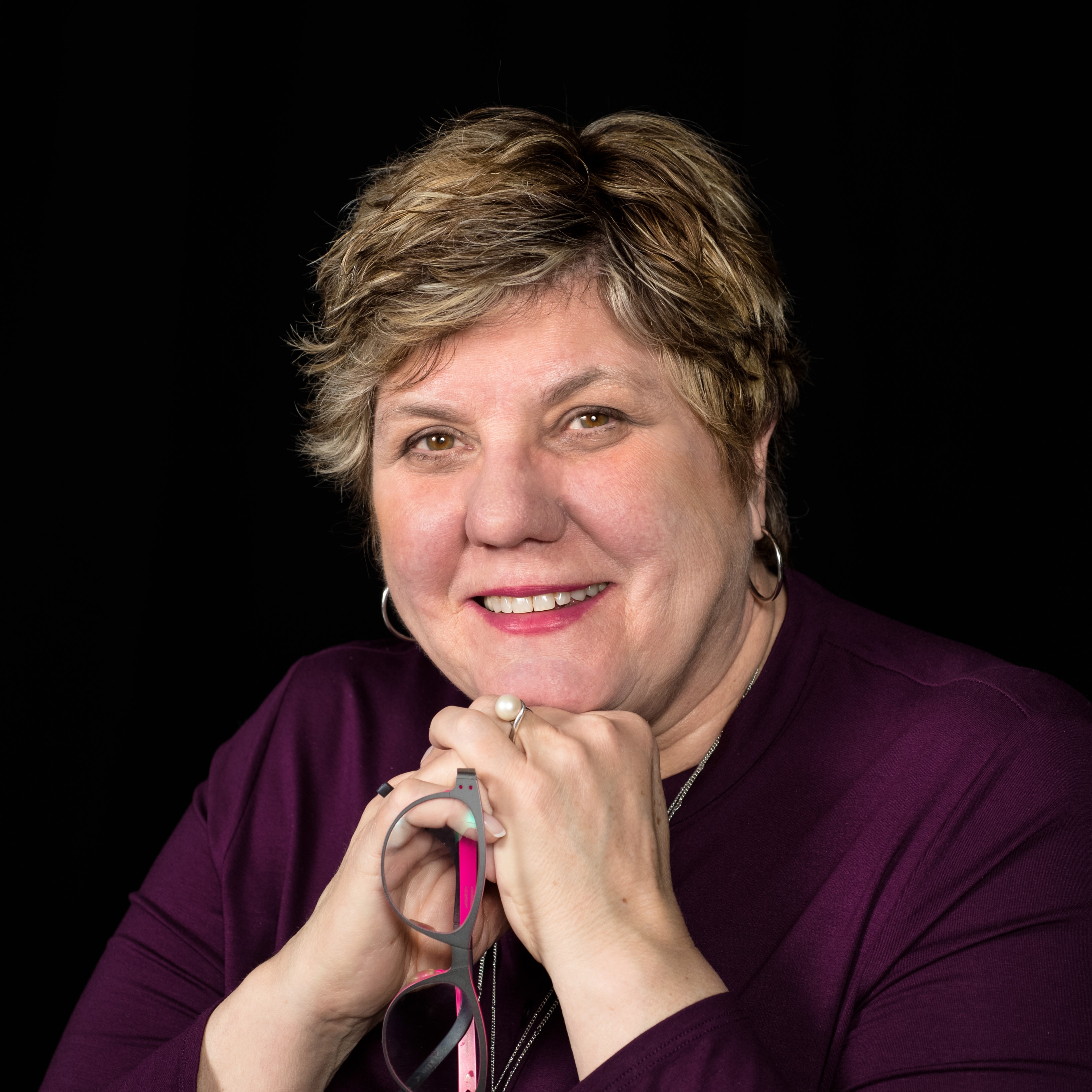
Patti Brennan
National Library of Medicine
Bio: Patricia Flatley Brennan, RN, PhD, is Director of the National Library of Medicine (NLM) at the National Institutes of Health. A leader in biomedical informatics and data science research, NLM also oversees a vast literature resources spanning 10 centuries, that includes print and electronic resources used billions of times each year by millions of people worldwide. Dr. Brennan has positioned NLM as an international hub of data science. NLM’s extensive data and information resources accelerate discovery, engage with new users, and advance the workforce for a data-driven future. Dr. Brennan has a Master of Science in Nursing from the University of Pennsylvania and a PhD in Industrial Engineering from the University of Wisconsin-Madison. Dr. Brennan is a member of the US National Academy of Medicine, holds fellowships in the American Academy of Nursing and the American College of Medical Informatics, and the American Institute for Medical and Biological Engineering.
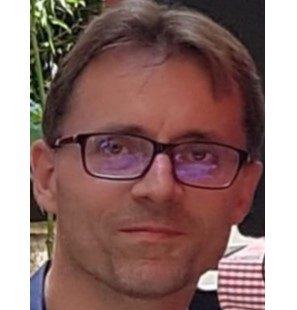
Alan Bridge
Swiss Institute of Bioinformatics
Expert curation at scale in UniProtKB/Swiss-Prot
Abstract: The UniProt Knowledgebase (UniProtKB)
is a comprehensive, high quality and freely accessible resource of protein
sequences and functional information. UniProtKB
is composed of an expert curated section, UniProtKB/Swiss-Prot, and its
automatically annotated
complement UniProtKB/TrEMBL (1). Expert curation of UniProtKB/Swiss-Prot focuses
on experimentally
characterized proteins of human origin and their homologs from closely related
species, as well as proteins of
biomedical relevance from plants, microbes, and viruses. It captures a broad
range of information on protein functions,
biological processes, cellular components, and molecular interactions, as well
as functional features such as active sites,
ligand-binding regions, and human variants with functional impact and their
links to disease, using a range of reference
vocabularies and ontologies including the Gene Ontology and many more, from
close to 250,000 literature references.
In this presentation, we will discuss the use of automated approaches to support
expert curation in UniProtKB/Swiss-Prot,
work performed with the Literature Search group of Dr Zhiyong Lu (NCBI). Deep
learning methods (2) use machine-readable
curated data on protein function and variation from UniProtKB/Swiss-Prot (3,4)
to automatically identify relevant literature
for expert curation. Additional filters that leverage UniProtKB and linked
datasets can then highlight literature describing
novel findings, such as new protein functions or biochemical reactions, or
findings linked to areas of active discourse,
such as the functional impact of variants whose clinical significance is
currently uncertain. Such text-mining approaches
will be crucial to focus, and more effectively scale, our expert curation effort
in UniProtKB.
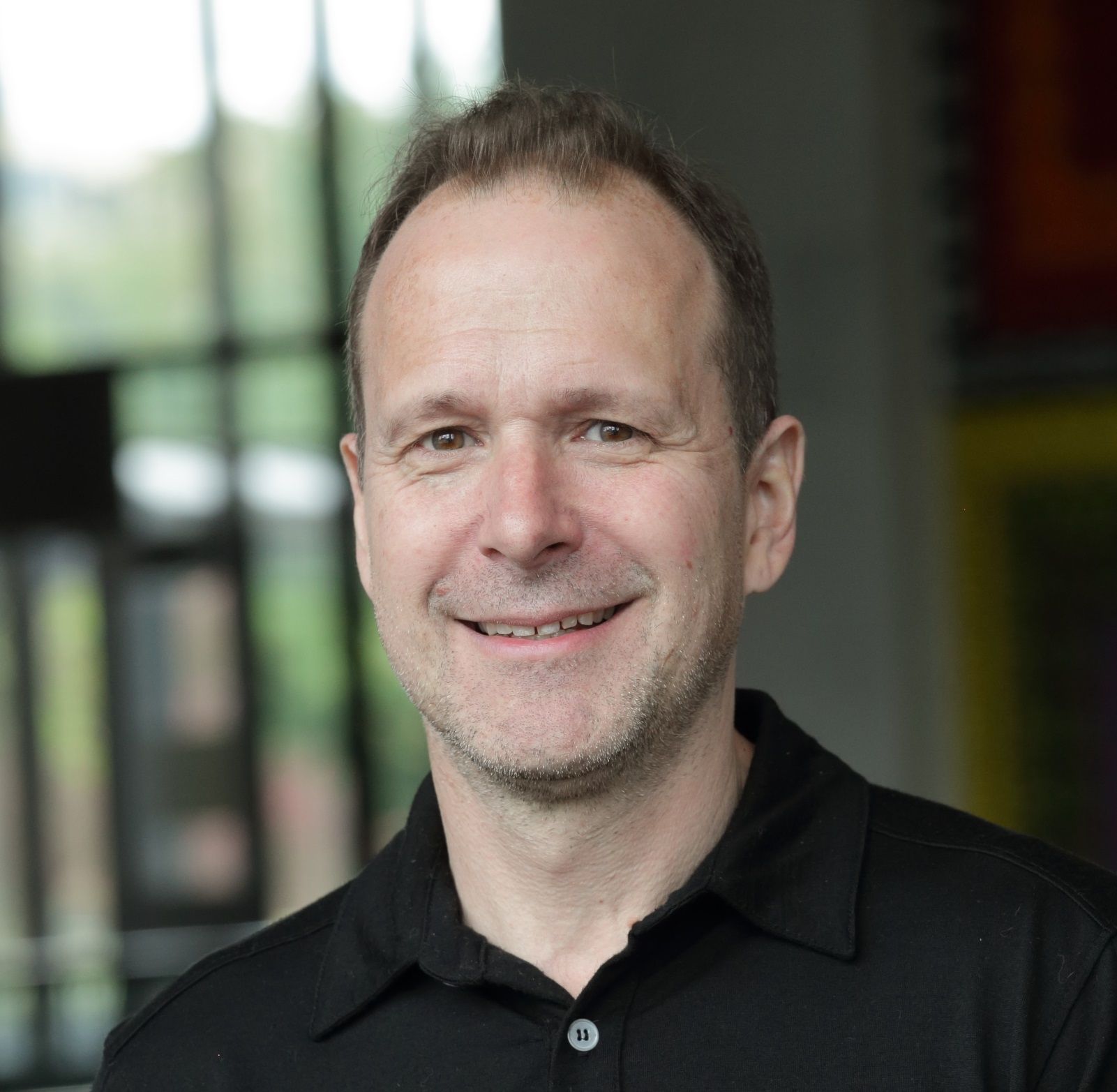
Rodney Brister
National Library of Medicine
Improving viral sequence data interoperability through targeted curation at scale
Abstract: The National Center for Biotechnology Information (NCBI) at the National Library of Medicine maintains two biological sequence archives, GenBank and the Sequence Read Archive (SRA). These open data repositories ingest data submitted independently by public health entities, research organizations, and individual scientists, aggregate and organize the data, and distribute it for use by the scientific community. Interoperability is critical to the usability and impact of data stored in these archives, and viral genome sequence data provides a case study in the curational processes necessary to support interoperability across datasets submitted by different providers over time. To improve data interoperability, NCBI viral sequence curation efforts have focused on three key areas - classification of sequences into the correct taxonomy nodes, genotypes, and lineages, sequence quality assessment and annotation, and normalization of descriptive metadata. Each of these focus areas requires similar outreach efforts with the scientific community and integration of interoperability goals into data curation flows. Typically, early curation processes emphasize manual operations, but implementation at scale ultimately requires automation. Ultimately these curation and data normalization efforts have enabled enhanced data search, retrieval, and data analysis and have impacted data across a large breadth of viruses, from bacteriophages to SARS-CoV-2.
Bio: J. Rodney Brister, Ph.D., is the Chief of NCBI Viral Resources and the Initiative Owner of the NCBI Cloud Data and Tools (STRIDES) and the NCBI TRACE SARS-CoV-2 Programs. Rodney received his Ph.D. in Molecular Genetics and Microbiology from the University of Florida where he defined the mechanism of Adeno-associated virus genome replication. He went on to describe the orchestration of bacteriophage T4 replication through multiple, genetically discrete origins of replication during post-doctoral work at NIDDK, NIH. Since joining NCBI, Rodney has been involved in several initiatives that support accessibility to sequence data through resources like NCBI Virus. These efforts include establishing community accepted data and metadata standards as well as the development and implementation of sequence data and metadata normalization processes. He is now building the foundation of a cloud dataverse based on next generations sequencing data and related sample metadata to meet public health needs and accelerate biomedical discoveries.
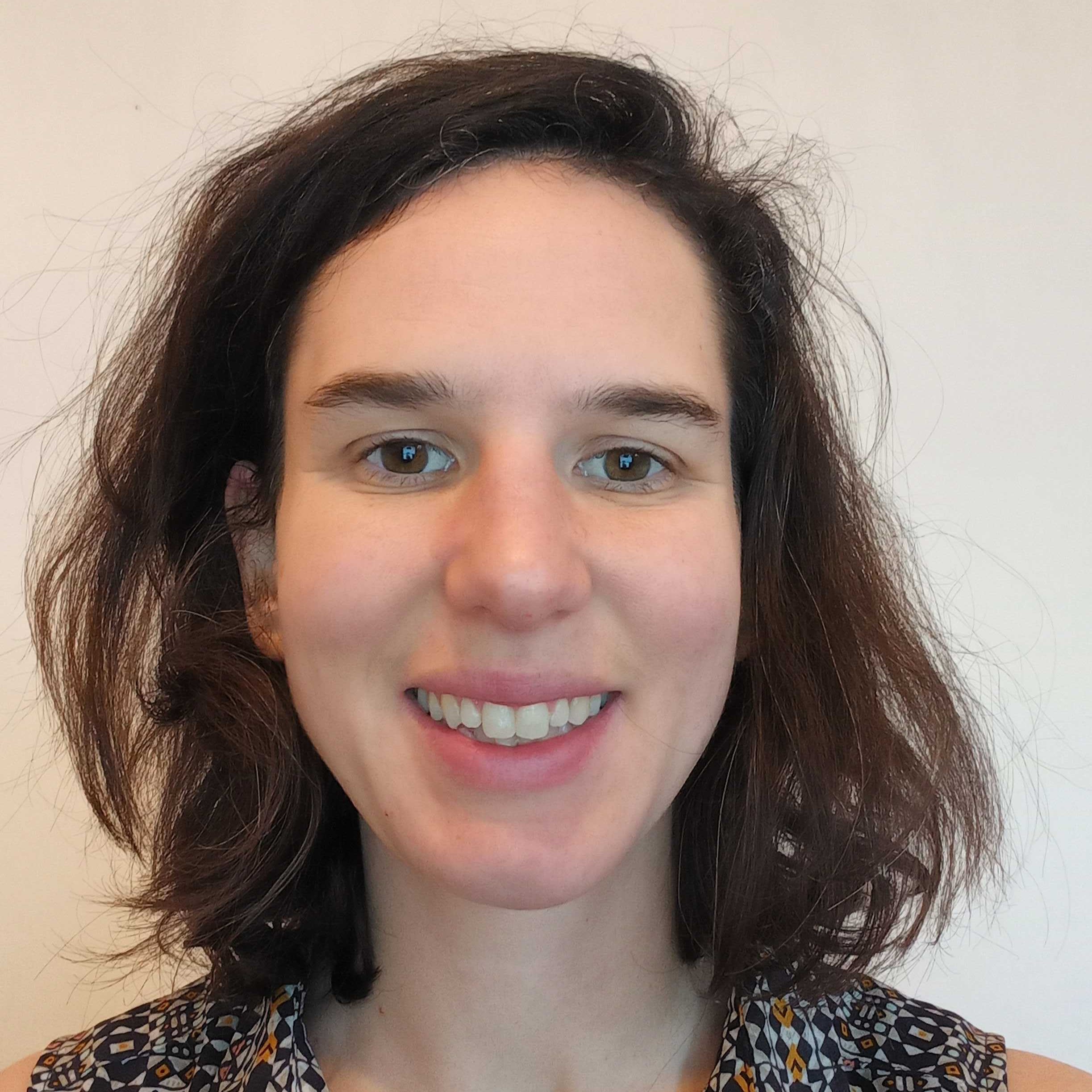
Lauren Cadwallader
Public Library of Science (PLOS)
Supporting Data Sharing in Scholarly Publishing
Abstract: There are various ways in which publishers can support and enable data sharing in scholarly publishing. PLOS introduced a mandatory data sharing policy in 2014 but our involvement as publishers does not end at creating policies. This talk will explore the issues of how publishers can support good practice in data sharing at a large scale and across multiple research disciplines, engaging peer reviewers with data availability and quality, and implementing a strong data availability policy. It will draw on the lessons learnt at PLOS journals and offer some reflections on how data sharing can be better supported within the scholarly ecosystem.
Bio: Dr Lauren Cadwallader is Open Research Manager at the Public Library of Science (PLOS). She is a scholarly communications professional, with experience in academia, libraries and publishing. She uses her expert knowledge of open research and skills in building relationships to drive forward the next stage in open research development in an organisation that is committed to the principles of openness and transparency.
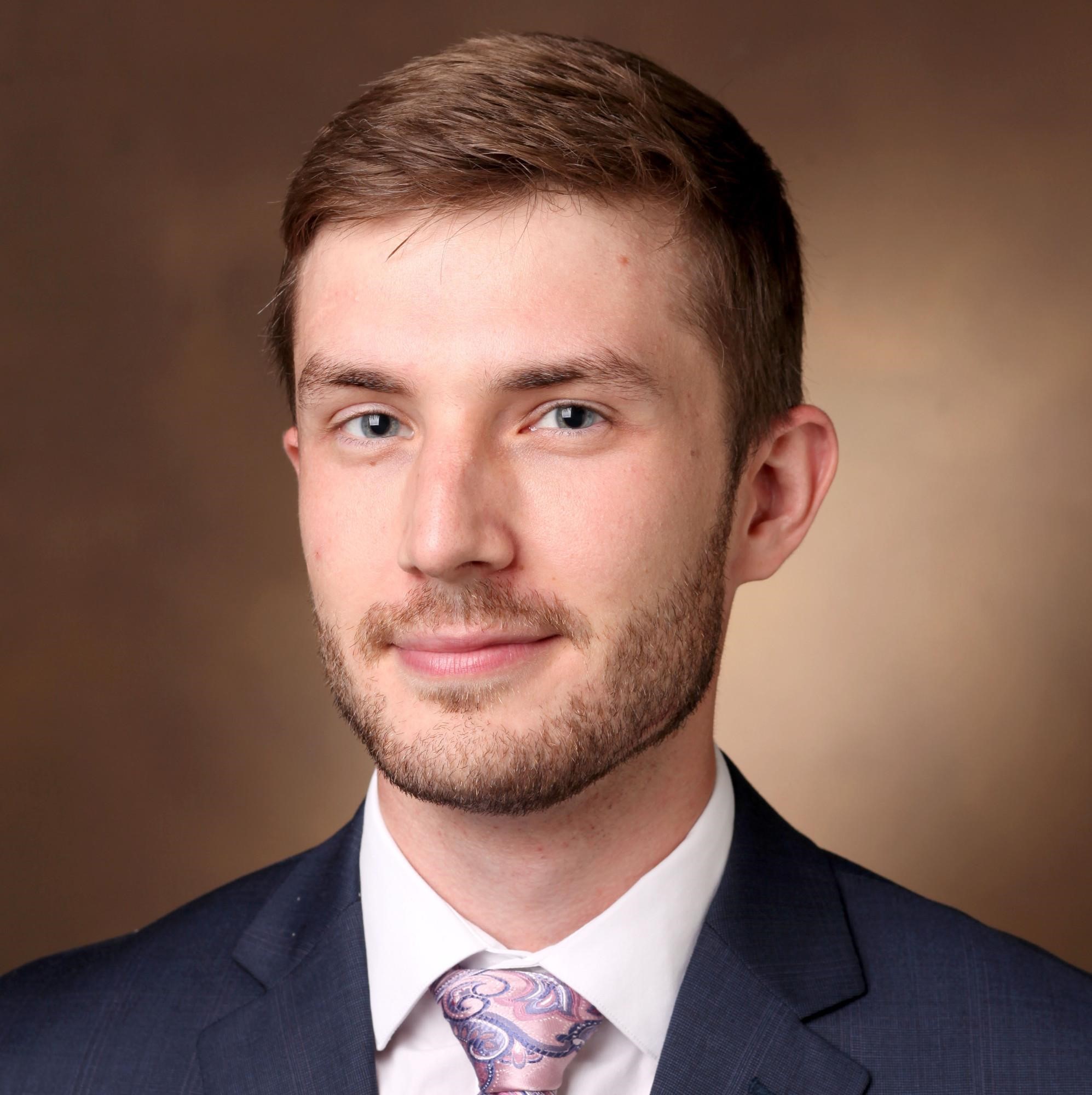
Robert Carroll
Vanderbilt University Medical Center
Curating Clinical and Phenotypic Data for the All of Us Research Program
The mission of the All of Us Research Program (AoURP), to accelerate health research and medical breakthroughs, enabling individualized prevention, treatment, and care for all of us, requires a robust environment for data capture, curation, and tools that support a diversity of participants, data, and researchers. Current data integrations include Participant Provided Information captured through web- and app-based surveys, Physical Measurements captured by trained AoURP staff in a web portal, and Electronic Health Record data uploaded by Health Provider Organizations. Future integrations include wearables, eg Fitbit, genomic data, and more. This presentation will cover the Data and Research Center and AoURP strategies for data intake, providing feedback on data quality, and curating data for research use. Particular focus will be given to the complexities of providing data quickly while ensuring reasonable data quality and supporting a broad set of research use cases and researcher skill sets.
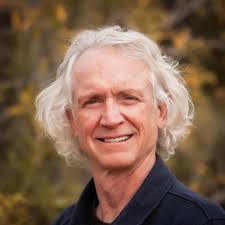
Mike Cherry
Stanford University
A philosophy and its implementation of these requirements for gold standard data and metadata
The Encyclopedia of DNA Elements (ENCODE) consortium conducts thousands of experiments each year on a variety of epigenomic assays. These data provide a reference set of functional non-coding elements in the human and mouse genome. To manage this volume of data programmatic submission is required. To maintain the highest possible standards for rich and accurate metadata we have deployed software to enforce standards. Even with automation we require several senior biocurators to assist data submitters and to shepherd the data before it can be released to the public. In this talk, I will discuss our philosophy on requirements for community gold standard large-scale genomics data, design of metadata models, and practical limitations of implementing this philosophy.
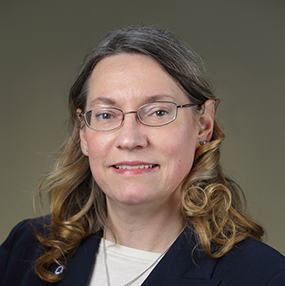
Susan Gregurick
National Institutes of Health (NIH)
Bio: Susan K. Gregurick, Ph.D., was appointed Associate Director for Data Science and Director of the Office of Data Science Strategy (ODSS) at the National Institutes of Health (NIH) on September 16, 2019. Under Dr. Gregurick’s leadership, the ODSS leads the implementation of the NIH Strategic Plan for Data Science through scientific, technical, and operational collaboration with the institutes, centers, and offices that comprise NIH. Dr. Gregurick received the 2020 Leadership in Biological Sciences Award from the Washington Academy of Sciences for her work in this role. She was instrumental in the creation of the ODSS in 2018 and served as a senior advisor to the office until being named to her current position.
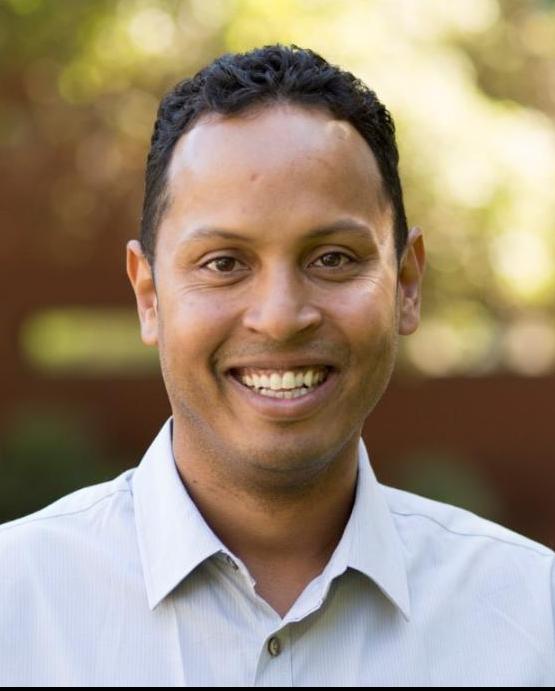
Dexter Hadley
University of Central Florida College of Medicine
From Curation to Community-Driven Artificial Intelligence
Abstract: Dr. Hadley will share his experience in developing artificial intelligence (AI) for clinical applications. Although commonplace today, electronic health records (EHRs) from hospitals are not readily machine readable. Moreover, privacy regulations (HIPAA) make it difficult to aggregate large-scale datasets required to achieve clinical-grade performance from state-of-the-art deep learning image classification algorithms. Dr. Hadley pioneered curation methods to automate assembly of routine clinical data into machine readable labeled imagesets to be used to predict patient outcomes on radiology. In collaboration with industry clinical partners, his laboratory develops an academic research data platform that allows patients to directly share their clinical EHR and imaging to be used to train, test, and validate clinical grade AI. In this short talk, he will discuss his community-driven approach to engage patients to share their data to predict clinical outcomes of breast cancer from mammography and COVID-19 outcomes from chest x rays.
Bio: Dr. Hadley’s research focus has long been geared towards translational medicine. He has made significant translational discoveries across the neuropsychiatric disease spectrum at the Center for Applied Genomics in The Children’s Hospital of Philadelphia, which houses the largest academic pediatric biobank in the world. Dr. Hadley’s work has resulted in an ongoing ‘precision medicine’ clinical trial for ADHD (ClinicalTrials.gov Identifier: NCT02286817) for a first-in-class, non-stimulant neuromodulator, and he co-founded a company, neuroFix Therapeutics, that was recently successfully acquired based on early positive trial results. At Stanford, Dr. Hadley moved towards developing tools and databases to organize public data stores by crowd-sourcing the annotation of over 1.6 million samples within NCBI’s Gene Expression Omnibus (GEO) for downstream analysis. As a PI at UCSF, Dr. Hadley’s laboratory integrated analysis of this datastore with many different functional databases and advances in digital health to better characterize signature patterns of disease and identify novel interventions to optimize disease management. Moving forward as a new PI at UCF jointly supported by HCA Healthcare, Dr. Hadley’s laboratory continues to build data driven interventions in healthcare that can be developed, tested, and eventually deployed at scale to improve the health and well-being of large populations of patients in a clinical setting.
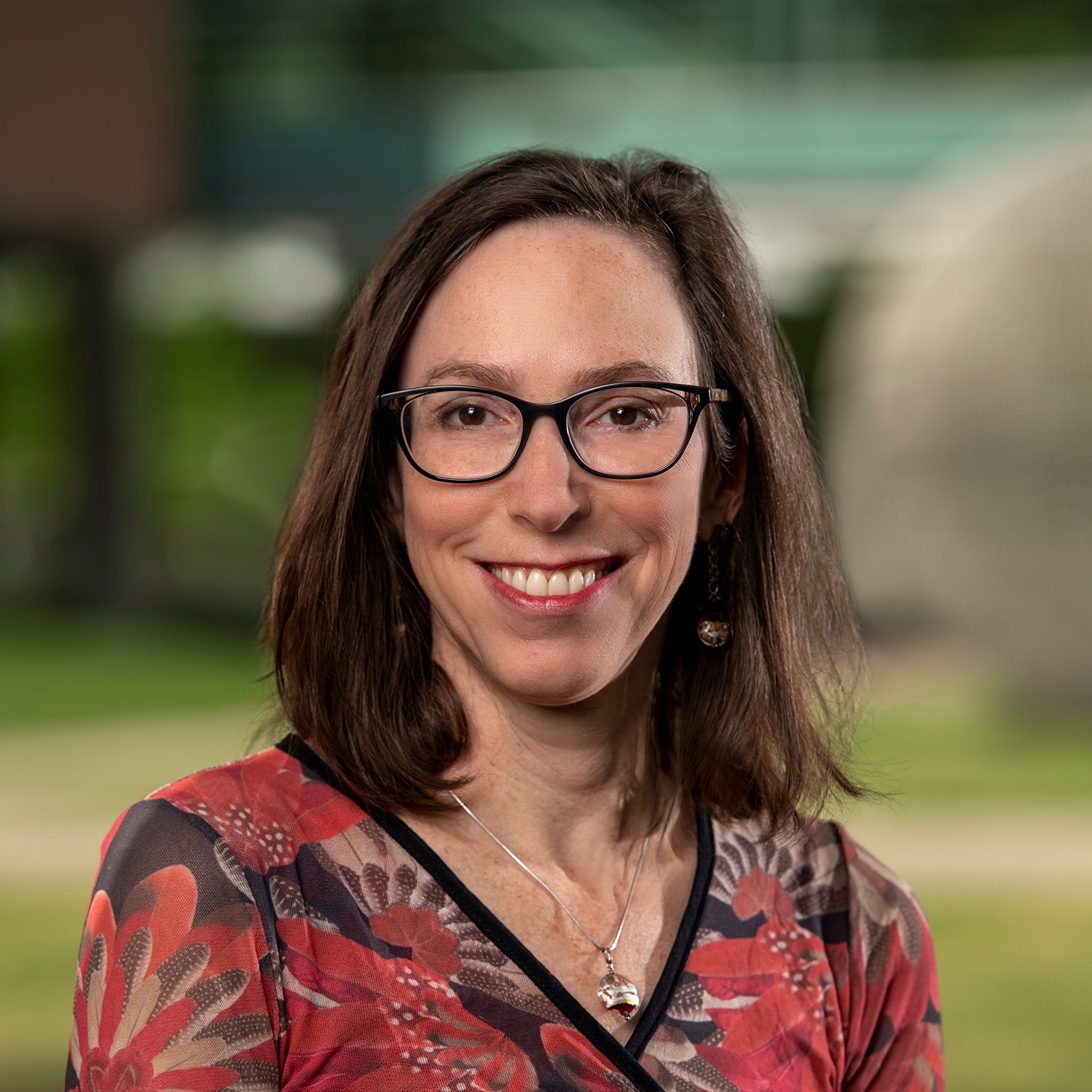
Melissa Haendel
University of Colorado School of Medicine
Curation pipelines at scale: how to realize enterprise-level interoperability
Abstract: Making data reusable for discovery and shared analytics is a laborious, specific-skill requiring task that most data providers do not have the resources, expertise, or perspective to perform. Equally challenged are the data re-users, who function in a landscape of bespoke schemas, formats, and coding - when they can get past understanding the licensing and access control issues. Making the most of our collective data requires partnerships between data providers and data consumers, as well as sophisticated strategies to address this myriad of issues. Furthermore, when transforming data to common models, there are data quality issues, errors, and a need to keep the transforms current. Such curation at scale means that the provenance of every transform needs to be recorded. All of the above is essentially moving careful manual curation and review to automation at enterprise scale. A robust dynamic relationship between those curating and automated systems and reports is also required. This talk will review different communities endeavors towards these ends from across the translational spectrum.
Bio: Melissa Haendel is the Chief Research Informatics Officer and Marsico Chair in Data Science at University of Colorado Anschutz Medical Campus, and the Director of the Center for Data to Health (CD2H). Her background is molecular genetics and developmental biology as well as translational informatics, with a focus over the past decade on open science and semantic engineering. Dr. Haendel’s vision is to weave together healthcare systems, basic science research, and patient-generated data through development of data-integration technologies and innovative data capture strategies. Dr. Haendel’s research has focused on integration of genotype-phenotype data to improve rare-disease diagnosis and mechanism discovery. She also leads and participates in international standards organizations to support improved data sharing and utility worldwide.
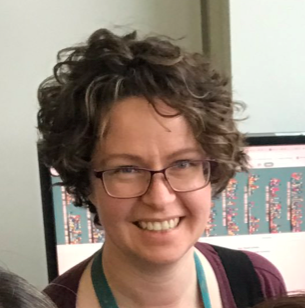
Laura Harris
The European Bioinformatics Institute
Scaling curation of variant-trait associations in the NHGRI/EBI genome-wide association studies Catalog (GWAS Catalog)
Abstract: The NHGRI-EBI GWAS Catalog is a central and comprehensive resource for the Genome Wide Association Study (GWAS) community, demonstrating the benefit of expert data curation and integration of full p-value GWAS summary statistics into a central repository for variant-trait associations. As of 1st October 2021, the Catalog contains curated results from 19,398 GWAS (100% increase from previous SAB report), comprising 293,040 variant-trait associations from 5,343 publications. Summary statistics content includes full p-value results from 9,622 published GWAS (521 publications, >30K files) and a further 1,252 pre-published datasets. The GWAS Catalog is now the largest aggregated international resource of FAIR (Findable, Accessible, Interoperable and Re-usable) GWAS data, and serves as a starting point for investigations to identify causal variants, calculate disease risk, understand disease mechanisms and establish targets for novel therapies. Summary statistics are used in meta-analyses, in follow-on analyses such as Mendelian randomisation, to generate polygenic scores, and in integration with other data types. The Catalog is highly used serving 220K users per annum via the web portal and 25M API calls. The Catalog is integrated with the Polygenic Score Catalog and shares processes and annotation standards enabling data flow and data integration.
Bio: Dr. Laura Wiseman Harris is Project Leader of the NHGRI-EBI GWAS Catalog. In this role, she co-ordinates the identification, curation, and release of GWAS Catalog data, working with software developers to improve user and curator interfaces, developing the GWAS Catalog's scientific content, user support, training, and outreach. Her background is in molecular biology and neuroscience, focusing on multi-omics analysis and data mining. Having spent many years using omics data to generate hypotheses and inform functional analyses, she understands the need for consistency, usability, and openness in data provision.
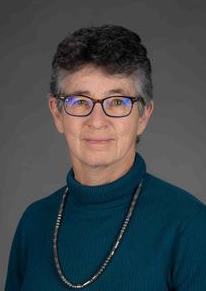
Lynette Hirschman
The MITRE Corporation
BioCreative – Assessing Text Mining Tools for the Biocuration Workflow
Abstract: BioCreative: Critical Assessment of Information Extraction in Biology is an international community-wide series of challenge evaluations focused on evaluating text mining and information extraction systems for the biological domain. Teams of molecular biologists, computer scientists and biocurators organize the evaluations creating “gold standard” resources from expert curated content. The evaluations address curation tasks from major biological databases, including, among others, UniProt, Comparative Toxicogenomics Database (CTD) and BioGRID. Applications have focused on triage and prioritization of literature; extraction and linking of bio-entities (e.g., genes, proteins, chemicals); and complex annotation tasks, such GO annotation or protein-protein interaction. BioCreative has also conducted user requirements analyses, developed interchange standards (BioC) and run experiments on persistent services. These activities have highlighted critical needs for automating the curation workflow, including access to the biological literature (paywalls, copyright and fair use, PDF based extraction); software engineering to facilitate insertion of tools into the curation workflow; adaptation of general tools to specific curation tasks; and design of appropriate human/machine interfaces to support high quality curation at scale.
Bio: Dr. Lynette Hirschman is a MITRE Technical Fellow and Chief Scientist for Biomedical Informatics at the MITRE Corporation in Bedford, MA, leading research at the intersection of biomedical informatics, computational biology, and natural language processing. Her research has been funded by DARPA, NSF, FDA and NIH. She received her Ph.D. from University of Pennsylvania in 1972 in mathematical linguistics, and worked at the New York University Linguistic String Project, Unisys Defense Systems, and the MIT Spoken Language Systems group before joining MITRE in 1993. She has published extensively in the fields of natural language processing, spoken language understanding, biomedical informatics, biocuration and evaluation of language understanding systems. She is a founder and co-organizer of BioCreative (Critical Assessment for Information Extraction in Biology), an international challenge evaluation for text mining in biology, and has been an active participant in the ISMB text mining community since 2003.
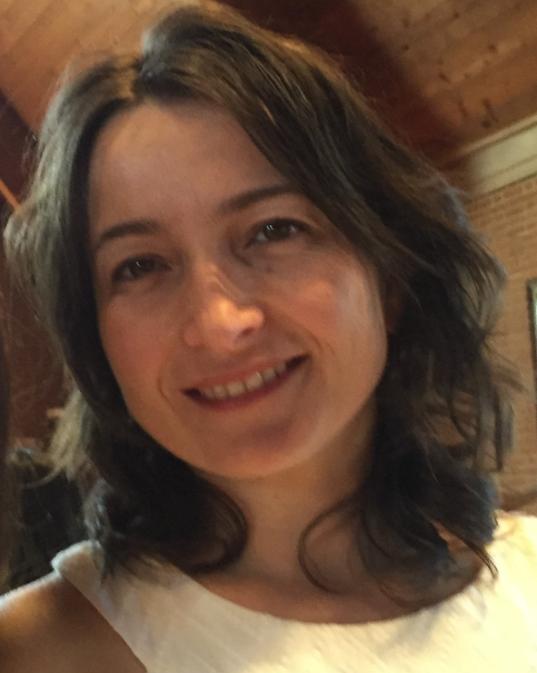
Rezarta Islamaj
National Library of Medicine
Enriching Gene and Chemical Links in Biomedical Literature
Abstract: Identification of relevant biomedical entities, such as genes and chemicals, is key to accurate article retrieval, classification, indexing and further understanding of the text. Furthermore, recognition of such entities helps ensure high-quality links between various NLM resources. Current Gene and Chemical NER tools have reached a plateau on their achievable accuracy. However, we can explore the power of deep learning technologies with more training data on a variety of model organisms, and more training data with full text annotations. To this end, we conducted two studies: NLM-Chem and NLM-Gene. NLM-Chem produced a corpus of 150 full text articles from the PubMed Central Open Access subset, doubly annotated for chemical entities by 10 expert NLM indexers. NLM-Gene produced a corpus of 550 PubMed articles, doubly annotated for gene entities from 6 expert NLM indexers. Both sets of articles were selected to be highly ambiguous, rich in biomedical entities, and covered 11 model organisms. We will discuss the challenges in corpora development, their use in developing newer, more accurate biomedical entity recognition tools, and their practical applications to increase the efficiency of Medline Indexing and of linking different NLM resources.
Bio: Dr. Rezarta Islamaj, Ph.D. in Computer Science from University of Maryland at College Park, is a Staff Scientist in the Computational Biology Branch of NCBI, NLM. She is a member of the Text Mining Research program where they are developing computational methods and software tools for analyzing and making sense of unstructured text data in biomedical literature and clinical notes towards accelerated discovery and better health. Her recent publications are focused on the following topics: Computer-assisted biomedical data curation, Biomedical named entity recognition and information extraction, Interoperability of data and tools, PubMed Search (e.g. author name disambiguation, understanding user needs for information retrieval). Dr. Rezarta Islamaj is an organizer on several community challenges such as BioCreative Workshops, aimed at promoting interoperability of data and tools, facilitating data sharing and text annotations for easier text mining in general, and coordinating curation efforts in developing lexical resources to facilitate better tool development for biomedical text mining.
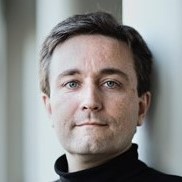
Lars Juhl Jensen
University of Copenhagen
Data and text mining of protein networks
Abstract: Methodological advances have in recent years given us unprecedented information on the molecular details of living cells. However, it remains a challenge to collect all the available data on individual genes and to integrate it with what is described in the scientific literature. The latest version of the STRING database aims to address this by consolidating known and predicted protein–protein association data across more than 5000 organisms. I will give an overview of the general approach we use to unify and score heterogeneous data for all evidence types, with particular focus on automatic text mining of associations from abstracts and full-text articles. Finally, I will briefly mention the EXTRACT curation tool, which is based on the same text-mining software.
Bio: Lars Juhl Jensen started his research career in Søren Brunak’s group at the Technical University of Denmark (DTU), from where he in 2002 received the Ph.D. degree in bioinformatics for his work on non-homology based protein function prediction. During this time, he also developed methods for visualization of microbial genomes, pattern recognition in promoter regions, and microarray analysis. From 2003 to 2008, he was at the European Molecular Biology Laboratory (EMBL) where he worked on literature mining, integration of large-scale experimental datasets, and analysis of biological interaction networks. Since 2009, he has continued this line of research as a professor at the Novo Nordisk Foundation Center for Protein Research at the Panum Institute in Copenhagen and as a founder, owner and scientific advisor of Intomics A/S. He is a co-author of more than 200 scientific publications that have in total received more than 30,000 citations. He was awarded the Lundbeck Foundation Talent Prize in 2003, his work on cell-cycle research was named “Break-through of the Year” in 2006 by the magazine Ingeniøren, his work on text mining won the first prize in the “Elsevier Grand Challenge: Knowledge Enhancement in the Life Sciences” in 2009, and he was awarded the Lundbeck Foundation Prize for Young Scientists in 2010.
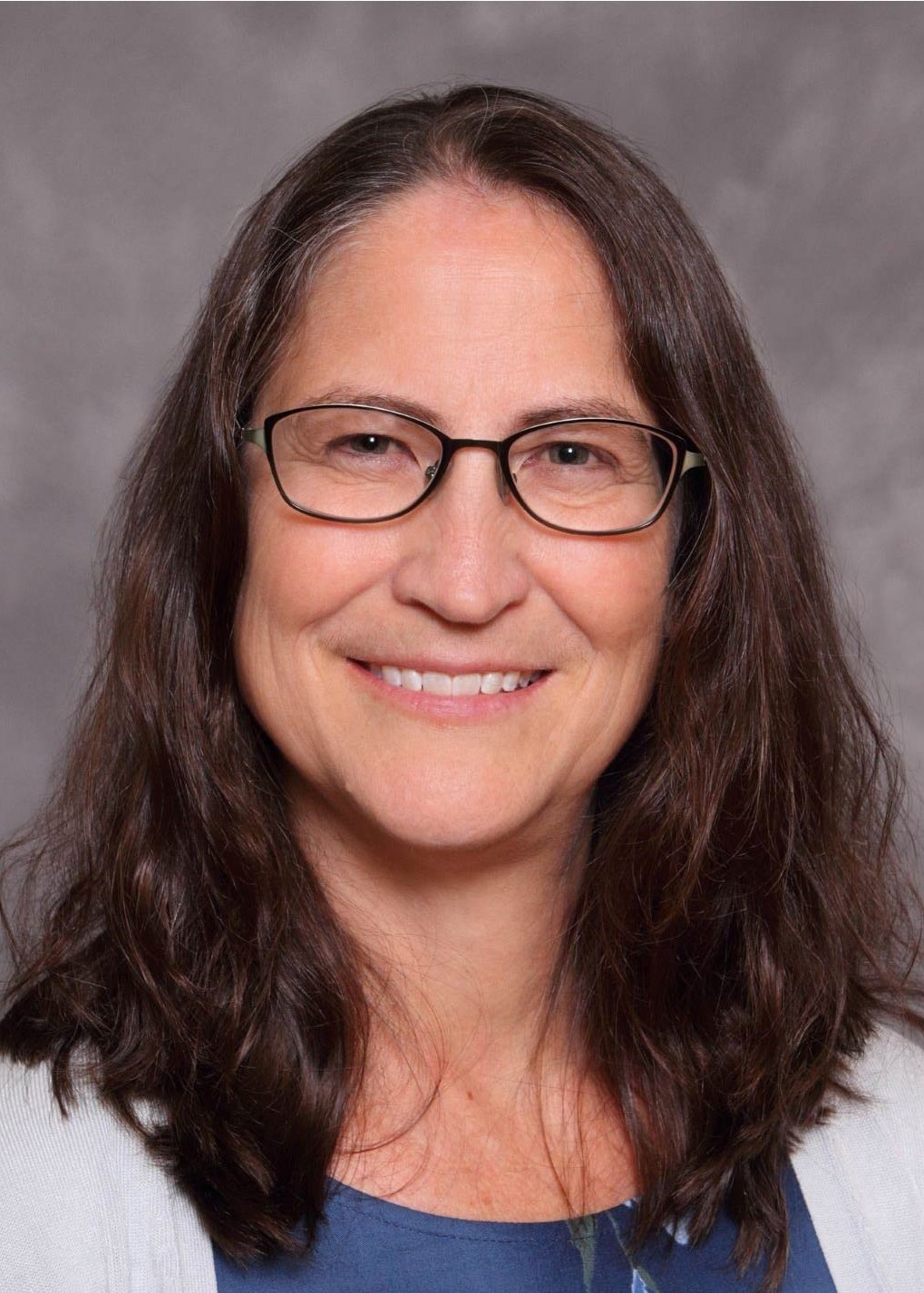
Anne Kwitek
Medical College of Wisconsin
Increasing Curation Efficiency Through Automation-Assisted Manual Curation
Abstract: The Rat Genome Database (RGD, https://rgd.mcw.edu) was developed with the goal of becoming a comprehensive resource for rat genetic, genomic, physiologic and disease data, and a platform for comparative studies across species. Recognizing the enormity of the task ahead—a search of PubMed for Rattus norvegicus returns over 1.7M articles—it was clear that curation efficiency would be paramount. Ongoing improvements in infrastructure, curation software and curation practices have resulted in concomitant improvements in curation efficiency.
Among these are:
• Curation software designed around RGD's curation workflow to streamline data entry and provide integrated QC, virtually eliminating the need to correct common entry errors.
• Automated pipelines that import data from outside sources and QC pipelines that find, and where possible correct, anomalies and errors with minimal curator oversight.
• OntoMate text mining software that tags abstracts with object symbols and names and ontology terms to assist curators with literature searching and triage. Embedding the tool into the curation software has facilitated the process of entering terms, objects and references for use in creating annotations.
• Data submission forms and spreadsheets to encourage standardization of user-submitted data at the time of submission.
As the number of species housed at RGD and the variety of data types increase, further enhancements only become more necessary. Since the bottleneck for RGD's curation workflow is finding relevant, curatable articles, RGD is committed to improving the OntoMate software. Work is underway to index full text articles. Access to object- and ontology-tagged full text has the potential to simplify the curator's tasks of determining usability of articles and finding the salient data in the text, figures and tables. Although challenges remain, the addition of automated processes and software has demonstrably increased RGD's curation efficiency.Bio: Dr. Kwitek is an animal and human geneticist whose research revolves around dissecting the genetic components of complex disease, with an emphasis on hypertension, diabetes, and the metabolic syndrome (MetS). In addition to animal and molecular genetics research, Dr. Kwitek has worked on developing knowledgebases and other resources for the laboratory rat and the scientific community. Dr. Kwitek also contributes to collaborative research projects that combine multiple genome-wide approaches to identify genes, pathways, and mechanisms involved in complex disease. Dr. Kwitek has spent over 25 years focusing on integrating genetics, genomics, and other ‘omics’ approaches to identify genes and mechanisms leading to complex disease using rat models and human populations, with an emphasis on hypertension, diabetes, and the metabolic syndrome (MetS). Dr. Kwitek’s research combines traditional genetic mapping and positional cloning with multiple genome-wide and systems biology approaches to identify genes, pathways, and mechanisms involved in complex disease. Dr. Kwitek’s laboratory expertise is in physiological studies in multiple rat models of cardiovascular disease and MetS, with extensive expertise in molecular and computational genetics, including genetic linkage analysis, high-throughput SNP genotyping, exome and transcriptome sequencing (bulk and single nucleus (sn)RNAseq)), and analyses including QTL, eQTL, network analyses, and comparative genomics. Dr. Kwitek was a coinvestigator on RGD from 1999-2007, 2019-2020, and principal investigator since 2020. Dr. Kwitek has been part of several NIH-funded large programs in addition to the Rat Genome Database, including the Alliance of Genome Resources, the Program for Genomic Applications, and the Somatic Cell Genome Editing Consortium, focusing on developing databases, visualization and analysis tools. I have also been part of multiple PPGs funded by NHLBI and NIAID.
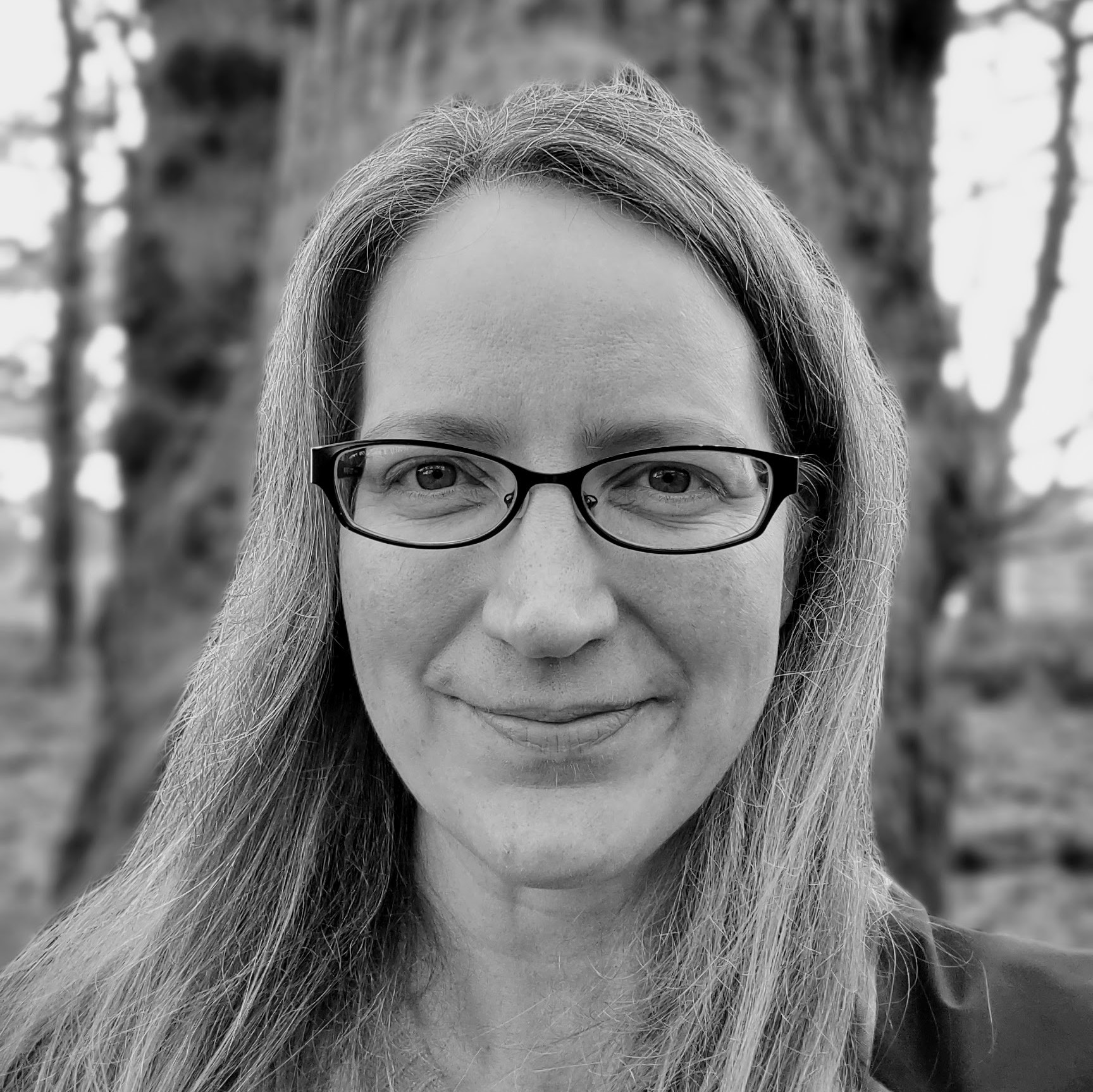
Allyson Lister
University of Oxford
FAIRsharing: curating an ecosystem of research standards and databases
Abstract: FAIRsharing is an informative and educational resource on interlinked standards, databases and policies, three key elements of the FAIR ecosystem. FAIRsharing is adopted by funders, publishers and communities across all research disciplines. It promotes the existence and value of these resources to aid data sharing and consequently requires a high standard of curation to ensure accurate and timely information is provided for all of our stakeholder groups. Here we discuss the methods employed and challenges faced during curation and maintenance of existing content as well as the introduction of new features. We will describe how our curation team uses a blend of manual and semi-automated curation to work on individual records and across large subsets of the registry. We also will discuss the benefits of both in-house curation and community-driven curation provided by our stakeholder groups.
Bio: Allyson is the FAIRsharing Coordinator for Content & Community at the University of Oxford, Department of Engineering Science. She is responsible for data curation and quality within FAIRsharing as well as for interactions and engagement with their community of users and stakeholders. She has a B.A. in Biology, moving into data science via a Master's degree in Bioinformatics from the University of York and a PhD in Computer Science from Newcastle University, where she studied data integration in the context of semantics and ontologies. She started her career at the European Bioinformatics Institute with the UniProt group, and has worked both for Newcastle University and the University of Manchester before moving to FAIRsharing in Oxford in 2015.
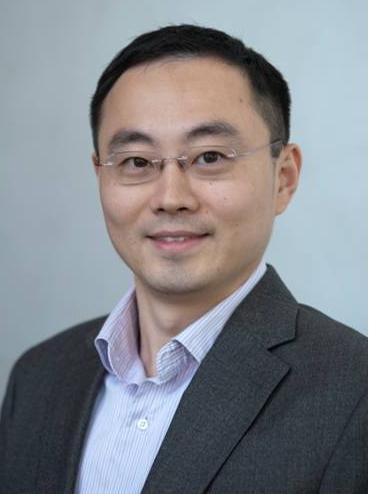
Zhiyong Lu
National Library of Medicine
LitVar: Literature Mining to Improve Variant Prioritization, Interpretation, and Curation
Understanding and assessing the associations of genomic variants with diseases or conditions and their clinical significance is a key step towards precision medicine. These tasks increasingly rely on accessing relevant manually curated information from existing domain databases. However, due to the sheer volume of medical literature and high cost of expert curation, curated variant information in existing databases are often incomplete and out-of-date. In response, we have developed LitVar (https://www.ncbi.nlm.nih.gov/research/litvar/) for the search and retrieval of standardized variant information in full-length articles. In addition, LitVar uses advanced text mining techniques to compute and extract relationships between variants and other associated entities such as phenotypes, diseases and drugs.
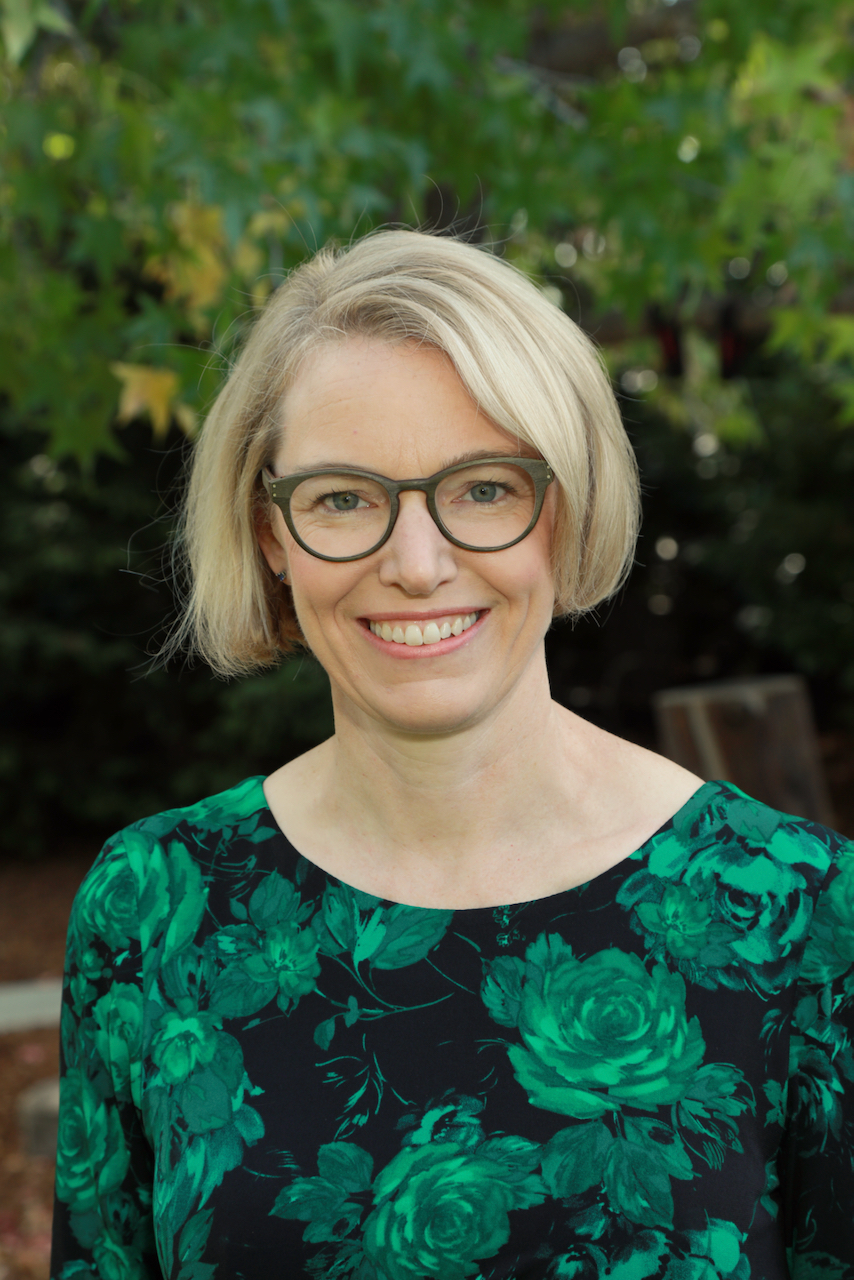
Alison Marsden
Stanford University
Automated Data Curation to Ensure Model Credibility in the Vascular Model Repository
Anstract:Three-dimensional anatomic modeling and simulation (3D M&S) in cardiovascular (CV) disease have become a crucial component of treatment planning, medical device design, diagnosis, and the FDA approval process. Comprehensive, curated 3-D M&S databases are critical to enable grand challenges, and to advance model reduction, shape analysis, and deep learning research for clinical application. However, large-scale open data curation involving 3-D M&S present unique challenges; simulations are data intensive, physics-based models are increasingly complex and highly resolved, and simulations require significant high-performance computing resources. Manually curating a large open-data repository, while ensuring the contents are verified and credible, is therefore intractable. Our work aims to overcome these challenges by developing broadly applicable automated curation data science to ensure model credibility and accuracy in 3-D M&S. In 2013, our team launched the Vascular Model Repository (VMR), providing 120 publicly-available datasets, including medical image data, anatomic vascular models, and blood flow simulation results, spanning numerous vascular anatomies and diseases. The VMR is compatible with SimVascular, the only fully open source platform providing state-of-the-art image-based blood flow modeling and analysis capability to the CV simulation community. In this talk, we will provide updates on several aspects of data curation and reliability related to the VMR including 1) automated methods for patient-specific blood flow simulation with reduced order 0D and 1D models, and 2) methods to extract uncertainty directly from medical images using Bayesian dropout networks. Finally, we will provide an overview and update on available community-wide open data and open source resources.
Bio: Dr. Marsden has over 15 years of experience developing novel methods for cardiovascular blood flow simulation and patient specific modeling. Application of our methods span congenital and acquired pediatric and adult cardiovascular disease, with emphasis on surgical and treatment planning, and mechanobiological factors important in disease progression. Dr. Marsden’s group has led recent developments in numerical methods and algorithms for multiscale modeling, fluid structure interaction, optimization, and uncertainty quantification. Dr. Marsden and her group developed and optimized a Y-graft design for the Fontan procedure in silico, which was translated to clinical use at two major centers and also introduced a novel approach for stage one single ventricle palliation. Dr. Marsden has substantial experience in multiscale hemodynamics simulation of cardiovascular disease. She has also developed a complete pipeline for uncertainty quantification in cardiovascular models, starting from uncertain clinical data, progressing to parameter estimation, and propagating through the model to generate statistics on output predictions. Ongoing projects include development of growth and remodeling methods to predict the progression of implanted tissue engineered vascular grafts (NIH funded), assessment of pulmonary replacement valve performance in Tetralogy of Fallot (AHA funded), multi-physics models of cardiac flow and mechanics (NSF funded), and development of models to study the onset and progression of vein graft failure after coronary artery bypass graft surgery (NIH funded). Dr. Marsden’s laboratory currently leads the SimVascular open source project, which has now attracted >5,000 users worldwide. A major goal of their work is the interaction of engineers with clinicians to develop quantitative and predictive simulation capabilities that advance medical science. Dr. Marsden’s track record in combining fundamental fluid mechanics and numerical methods with clinical applications in cardiovascular disease has uniquely positioned her as a leader in the interdisciplinary field of computational modeling of cardiovascular biomechanics.
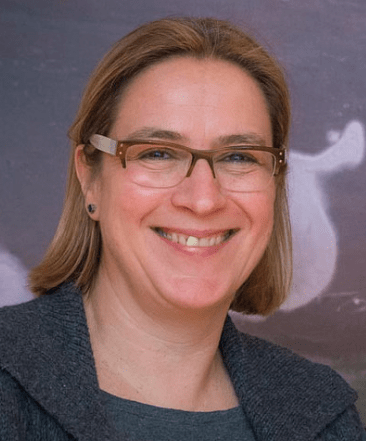
Jo McEntyre
EBI – The European Bioinformatics Institute
Shared infrastructure to support curation workflows at Europe PMC
Europe PMC is a database of full text research articles and abstracts, built in collaboration with PMC at the NLM, NIH. We have developed an annotations infrastructure based on open access full text articles and abstracts in Europe PMC, in which any text mining or curation group can contribution annotations. Submissions to the annotations databases () need to adhere to a json data format and can be uploaded dynamically via APIs, or in batch mode. The system currently contains annotations from about twelve text mining and curation groups, over a 1B annotations in total, covering entity recognition of genes/proteins, diseases, organisms, mutations, dataset and data resource citations, cell lines and drugs, and some relationships such as genes-diseases. All annotations are shared for reuse via APIs, and viewable in Europe PMC web pages. We intend that this community effort to provide such a broad set of annotations will be used increasingly in curation workflows.
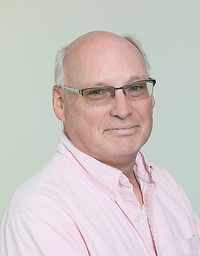
Jim Mork
National Library of Medicine
NLM Curation using the Medical Text Indexer
Abstract: The MEDLINE Curation process at the U.S. National Library of Medicine (NLM) continually evolves to meet the ever-increasing demands, user needs, and process driven goals. The NLM Medical Text Indexer (MTI) is one part of this curation process that has been designed to help the human indexers at NLM curate more efficiently and consistently. We will look at the Medical Subject Heading (MeSH) controlled vocabulary used in the curation process, how MTI fits into this process, and how we are looking at automating parts of the curation process to better utilize the human indexers. We will also look at some of the challenges being faced by the curation process, like trying to make a computer program understand what “falling risks” might mean in various contexts.
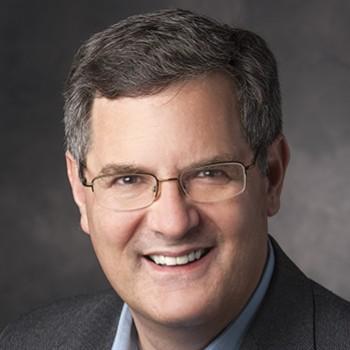
Mark Musen
Stanford University
Technology is not enough. Curation at scale will require mobilizing scientific communities—at scale
Abstract: The FAIR guiding principles are largely propositions about metadata, requesting, among other things, that metadata be “rich” and that they “adhere to community standards.” Data can be FAIR only when there are community standards for metadata schemas and for discipline-specific ontologies. When such standards exist, tools such as CEDAR can help investigators to author rich and standards-adherent metadata when their datasets are first assembled. Once the datasets have been uploaded to a repository, our “Metadata Powerwash” acts as a kind of spellchecker to transform legacy metadata to better comply with community standards. Both these technologies, however, assume that community standards are available in the first place. For most areas of science, however, standards are weak or nonexistent. Curation at scale will require massive efforts to encourage scientific communities to develop the standards needed to drive the underlying technologies. There is much work to be done by professional societies, investigators, journals, and funders to bring about the cultural change needed to make curation technology maximally successful and to make scientific datasets FAIR.
Bio: Dr. Musen is Professor of Biomedical Informatics and of Biomedical Data Science at Stanford University, where he is Director of the Stanford Center for Biomedical Informatics Research. Dr. Musen conducts research related to open science, intelligent systems, computational ontologies, and biomedical decision support. His group developed Protégé, the world’s most widely used technology for building and managing terminologies and ontologies. He served as principal investigator of the National Center for Biomedical Ontology, one of the original National Centers for Biomedical Computing created by the U.S. National Institutes of Heath (NIH). He directs the Center for Expanded Data Annotation and Retrieval (CEDAR), founded under the NIH Big Data to Knowledge Initiative. CEDAR develops semantic technology to ease the authoring and management of biomedical experimental metadata. Dr. Musen directs the World Health Organization Collaborating Center for Classification, Terminology, and Standards at Stanford University, which has developed much of the information infrastructure for the authoring and management of the 11th edition of the International Classification of Diseases (ICD-11).
Dr. Musen was the recipient of the Donald A. B. Lindberg Award for Innovation in Informatics from the American Medical Informatics Association in 2006. He has been elected to the American College of Medical Informatics, the Association of American Physicians, the International Academy of Health Sciences Informatics, and the National Academy of Medicine.
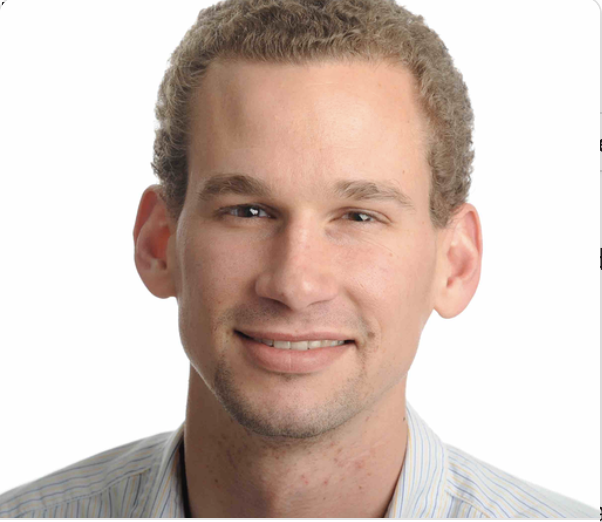
Eric Nawrocki
National Library of Medicine
Automated validation and annotation of SARS-CoV-2 sequences for GenBank using VADR
Abstract: Throughout the coronavirus pandemic, the number of SARS-CoV-2 sequences submitted to GenBank grew from several hundred per month in spring 2020 to tens of thousands per month by spring 2021. To validate and annotate these sequences we adapted our software package VADR by making it faster and by improving its ability to distinguish problematic sequencing and assembly artifacts from expected natural sequence variation in SARS-CoV-2 sequences.
Bio: Eric received his Ph.D. from Washington University in St. Louis under adviser Dr. Sean Eddy. His research focuses on software and algorithm development for biological sequence analysis, particularly for non-coding RNAs and on viral genome annotation. In 2015, he started as a staff scientist at the National Center for Biotechnology Information (NCBI) and has developed the Ribovore software package for ribosomal RNA sequence analysis and the VADR software package for viral genome validation and annotation.
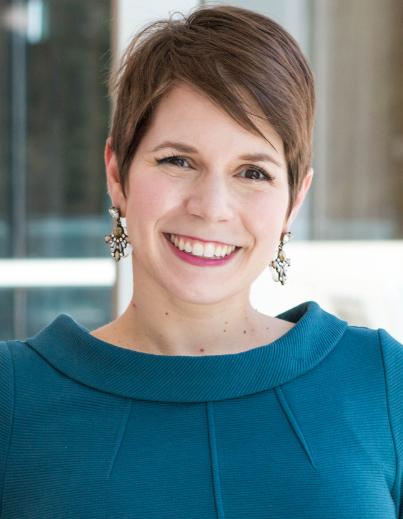
Erin Riggs
Geisinger Health System
ClinGen: Utilizing Volunteer Efforts to Curate the Clinical Genome
The Clinical Genome Resource (ClinGen) is an NIH-funded initiative dedicated to identifying genes and variants of clinical relevance for use in precision medicine and research. ClinGen uses publicly available data to answer a number of different curation questions, including: Which genes are associated with disease (gene-disease validity)? Which variants within those genes actually cause disease (variant pathogenicity)? Which genes or genomic regions cause disease via haploinsufficiency or triplosensitivity (dosage sensitivity)? Could the disease be prevented or mitigated if the risk were known (clinical actionability)? Since 2013, ClinGen curators have evaluated over 870 gene-disease associations, the pathogenicity of over 10,000 sequence variants, the dosage sensitivity of over 1400 genes/genomic regions, and the clinical actionability of over 125 outcome-intervention pairs. Currently, curating the clinical genome in this manner requires significant manual. In late 2018, ClinGen launched its volunteer program, soliciting members of the genomics community to volunteer their efforts to bolster curation activities. Since that time, over 350 individuals have volunteered to curate with ClinGen. Training for each of our curation activities occurs on a quarterly basis, and consist of a mixture of self-guided and “live” activities. The availability of additional curation volunteers is both increasing the throughput of our curation groups and uncovering new bottlenecks, requiring ClinGen to consider alternate approaches, including restructuring meetings/evaluation processes, and piloting efforts to leverage manual data annotation processes to support machine learning. ClinGen strives to maintain a balance between curation speed and quality, and aims to utilize both machine learning and manual approaches to maximize efficiency.
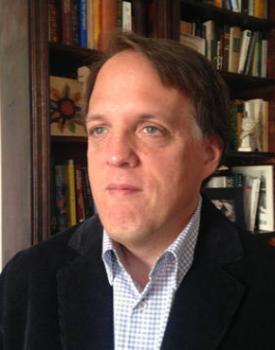
Peter Robinson
The Jackson Laboratory for Genomic Medicine
The GA4GH Phenopacket schema: A computable representation of clinical data for precision medicine
Abstract: Despite great strides in the development and wide acceptance of standards for exchanging structured information about genomic variants, there is currently no corresponding standard for exchanging phenotypic data, which has impeded sharing phenotypic information for computational analysis. This standards gap, with the corresponding paucity of phenotypic data in genomics studies, is increasingly problematic for numerous applications such as disease diagnostics, mechanism discovery, and treatment development. Here, we introduce the Global Alliance for Genomics and Health (GA4GH) Phenopacket schema, which supports global exchange of computable case-level phenotypic information for all types of disease diagnosis and research, including for infectious disease, cancer, and rare disease. Phenopackets are designed to be used across a comprehensive landscape of applications including diagnostic tools, Electronic Health Record systems, biobanks, biomedical knowledge bases, the scientific literature, medical case reports, patient registries, patient self-reporting tools and surveys, patient data aggregation (“matchmaking”), and clinical diagnostic laboratories. The Phenopacket schema is a freely available, community-driven standard that streamlines exchange and systematic use of phenotypic data, which will facilitate sophisticated computational analysis of both clinical and genomic information to help improve our understanding of diseases and our ability to manage them. The Phenopacket schema provides a foundation for the curation of case reports from medical journals in addition to many other envisaged applications.
Bio: Peter Robinson is a Professor of Computational Biology at The Jackson Laboratory for Genomic Medicine, Farmington, CT. Prior to his relocation in August 2016, Peter was a “W2” Professor for Medical Genomics at the Charité Universitätsmedizin Berlin, which is the largest academic hospital in Germany and was adjunct professor for Bioinformatics in the Department of Mathematics and Computer Science of the Free University of Berlin, one of Germany’s leading research universities. Peter has degrees in Mathematics, Medicine, and Computer Science, and has practiced as a pediatrician in Berlin, where he had full certification as a Pediatrician as well as a “Habilitation” in human genetics (roughly: a recognition of research achievements similar to a PhD as well as a formal qualification to teach a subject at a German university). Peter’s research career initially involved molecular genetics projects supported by the Deutsche Forschungsgemeinschaft (DFG) and the European Commission’s FP7 program. His research group initially characterized Mendelian disease-associated genes including CA8 and PIGV, characterized a novel mode of pathogenesis in a mouse model of Marfan syndrome, for which we successfully tested a proof of principle therapeutic concept. The central research theme of Peter’s research since 2004 has been the development of computational resources and algorithms for the study of human disease; he has exploited knowledge of clinical medicine, molecular genetics and computer science to develop the Human Phenotype Ontology (HPO), which provides comprehensive bioinformatic resources for the analysis of human diseases and phenotypes, offering a computational bridge between genome biology and clinical medicine. The HPO has been adopted by major international projects such as the NIH Undiagnosed Diseases Network and Genomic England’s 100,000 Genomes project and has become the de facto standard for computational phenotype analysis in rare disease. The HPO is being developed as a team-science project within the Monarch Initiative. Peter’s group develops algorithms for the analysis of diagnostic exome and genome data and statistical methods for the analysis of genomic data including ChIP-seq, RNA-seq, and Capture Hi-C.
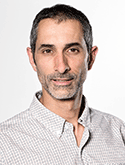
Patrick Ruch
University of Applied Sciences Geneva
Text Mining to Support the Curation and Interpretation of Clinically-relevant Variants
The Swiss Variant Interpretation Platform for Oncology is a centralized, joint and curated database for clinical somatic variants piloted by a board of Swiss healthcare institutions and operated by the SIB Swiss Institute of Bioinformatics. To support this effort, SIB Text Mining designed a set of text analytics services. The presentation focuses on three of those services. First, the automatic annotations of the literature with a set of terminologies has been performed, resulting in a large annotated version of MEDLINE and PMC. Second, a generator of variant synonyms for single nucleotide variants has been developed using publicly available data resources, as well as patterns of non-standard formats, often found in the literature, and useful to support the recall of the search engine, in particular for variants or unknown or quasi-unknown significance. Third, a literature ranking service enables to retrieve a ranked set of MEDLINE abstracts given a variant and optionally a diagnosis. The annotation of MEDLINE and PMC resulted in a total of respectively 785,181,199 and1,156,060,212 annotations, which means an average of 26 and 425 annotations per abstract and full-text article. The generator of variant synonyms enables to retrieve up to 42 synonyms for a variant. The literature ranking service reaches a precision (P10) of 64%, which means that almost two thirds of the top-10 returned abstracts are judged relevant. Further services will be implemented to complete this set of services, such as a service to retrieve relevant clinical trials for a patient and a literature ranking service for full-text articles.
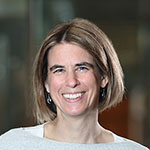
Valerie Schneider
National Library of Medicine
The impact of SARS-CoV-2 on the NCBI curation landscape
Abstract: NCBI is host to a wide range of publicly accessible databases and tools for biomedical sequences and literature. With more than 33 million citations in PubMed and 900 billion base pairs in GenBank, curation at scale has long been an essential element in managing this information. This presentation will discuss how existing curation processes, such as those for checking sequence quality and taxonomy, standardized annotation, and normalization, were applied and modified to address the influx of SARS-CoV-2 sequence data. In addition, it will look at new curation activities instantiated for SARS-CoV-2 associated literature and public health resources and their applicability to other content. Finally, it will examine how, in both cases, the need for a fast response to the emergent situation required a mix of automated and manual processes, and how that balance evolved over the course of time.
Bio: Valerie Schneider, Ph.D., is the deputy director of Sequence Offerings and the head of the Sequence Plus program in the Information Engineering Branch at NCBI. In these roles, she coordinates efforts associated with the curation, enhancement, and organization of sequence data, as well as oversees tools and resources that enable the public to access, analyze, and visualize biomedical data. She also manages NCBI’s involvement in the Genome Reference Consortium, the international collaboration tasked with maintaining the value of the human reference genome assembly.
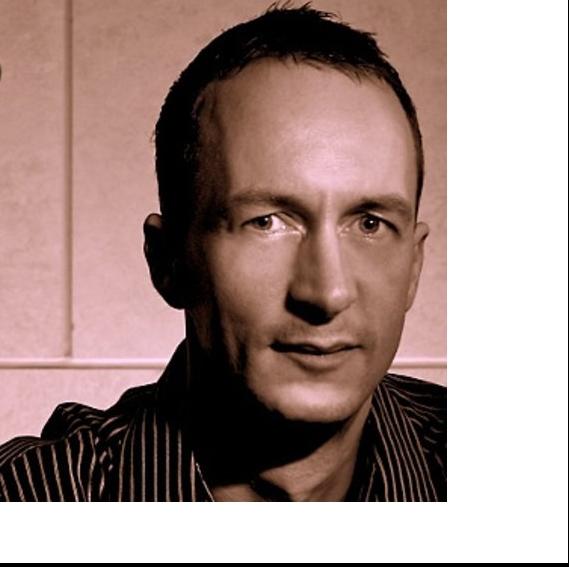
Stephan Schürer
University of Miami
Novel Tools to Enable FAIR and Open Data
Abstract: As part of several national research consortia, namely the Library of Integrated Network-based Cellular Signatures (LINCS), Big Data to Knowledge (BD2K), Illuminating the Druggable Genome (IDG), and Radical Acceleration of Diagnostics (RADx), we have developed data standards, ontologies, and software tools to harmonize, annotate, and formally describe diverse biological data types to support the research community’s goals of making these digital resources Findable, Accessible, Interoperable, and Reusable (FAIR). Such data include multi-omics datasets, chemical biology data, biochemical target data, chemical structures, cell lines, proteins and more. To standardize and integrate these datasets we leverage ontologies including BioAssay Ontology (BAO), Drug Target Ontology (DTO), Cell Line Ontology (CLO), which we developed or co-developed. BAO was recently expanded significantly to include pharmacokinetics-, pharmacokinetics-, and drug safety assays. DTO was expanded to include the majority of druggable proteins. To enable FAIR data in practice, we have developed and implemented infrastructure and processes to handle the end-to-end data management pipeline from receiving data and metadata, registration, annotation, mapping to external reference resources, and publication, implemented in software tools such as the Resource Submission System (RSS, https://rss.ccs.miami.edu/) and the LINCS Data Portal (LDP, http://lincsportal.ccs.miami.edu/) of the IDG and LINCS programs, respectively. We are also developing better tools to support FAIR data curation leveraging ontologies. OntoloBridge (https://ontolobridge.ccs.miami.edu/) facilitates the interaction and coordination of ontology users and -maintainers to request and process novel ontology terms. In combination, data standards, ontologies, and research data management software tools enable FAIR open data that drive scientific research.
Bio: Dr. Schurer is a Professor in the Department of Molecular and Cellular Pharmacology at the University of Miami and Program Director of Drug Discovery at the Center for Computational Science (CCS) and. With training in synthetic organic chemistry, he has 15 years of research and management experience in industry and academia working on data-standards, -integration, -modeling, scientific content and software development, chemo- and bioinformatics. Over the years, Dr. Schurer has been involved in many small molecule probe and drug discovery projects and has worked in leadership positions in several national multi-site research consortia including the NIH Molecular Libraries Program, the Library of Integrated Network-based Cellular Signatures (LINCS), Big Data to Knowledge (BD2K), and the Illuminating the Druggable Genome (IDG) projects. At the Scripps Research Institute in Florida and the University of Miami, Dr. Schurer implemented operational informatics and research cheminformatics capabilities to support early stage drug discovery screening and optimization projects. Previous to working in academia, Dr. Schurer directed groups of scientists to develop scientific content products, which are licensed to pharmaceutical and biotechnology companies. Dr. Schurer’s research is focused on developing solutions for large-scale integration and modeling of systems biology ‘omics’ and molecular simulations of drug protein interaction data to develop novel targeted small molecules. Dr. Schurer’s research group applies distributed and parallelized bio- and chemoinformatics tools and builds modeling pipelines to predict small molecule properties and targets, drug mechanism of action, –specificity, –promiscuity and –polypharmacology. To physically make and test the most promising small molecules, they develop computationally-optimized synthetic routes and applies parallel synthesis technologies to synthesize small compound libraries. Together these capabilities enable efficient prioritization of novel drug target hypotheses, identification of precision drug combinations, and the development of novel small molecule tool compounds as starting points for drug discovery projects.
https://rss.ccs.miami.edu/
http://lincsportal.ccs.miami.edu/dcic-portal/
https://ontolobridge.ccs.miami.edu/
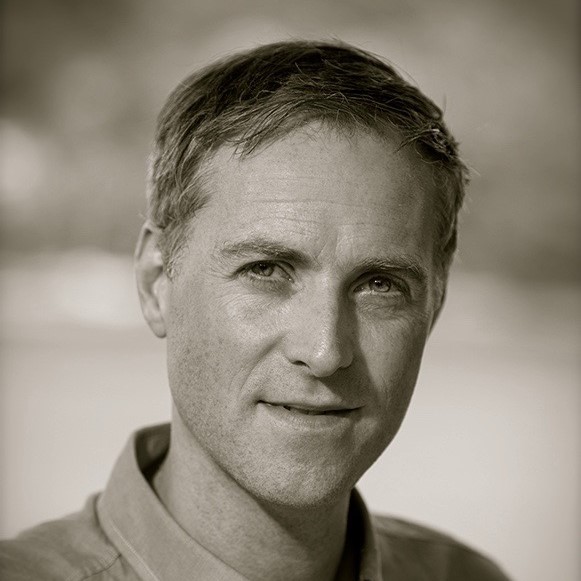
Richard Sever
bioRxiv and Cold Spring Harbor Laboratory
Curating Preprints
Abstract: bioRxiv and medRxiv post hundreds of preprints every week, across the entire spectrum of biomedical research. Efforts that allow readers to search for, discover and filter preprints of interest are therefore critical. The bioRxiv and medRxiv servers provide a variety of discovery and alerting tools. They also integrate with numerous third-party curation efforts, including automated services, journals and groups of academics who select and rapidly review content in specific areas. These interactions provide a model for an open, decoupled ecosystem for scientific communication.
Bio: Richard Sever, PhD is Assistant Director of Cold Spring Harbor Laboratory Press at Cold Spring Harbor Laboratory in New York and Co-Founder of the preprint servers bioRxiv and medRxiv. He also serves as Executive Editor for the Cold Spring Harbor Perspectives and Cold Spring Harbor Protocols journals and launched the precision medicine journal Cold Spring Harbor Molecular Case Studies. After receiving a degree in Biochemistry from Oxford University, Richard obtained his PhD at the MRC Laboratory of Molecular Biology in Cambridge, UK. He then moved into editorial work, first as an editor at Current Opinion in Cell Biology and later Trends in Biochemical Sciences. He subsequently served as Executive Editor of Journal of Cell Science, before moving to Cold Spring Harbor Laboratory in 2008.
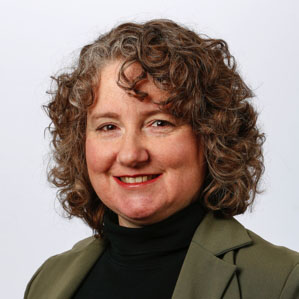
Cynthia Smith
The Jackson Laboratory
Advances in Literature Curation at Mouse Genome Informatics
Abstract: Although the numbers of yearly accessions to PubMed are rapidly rising, the genetics and genomics literature identified as relevant for curation into the Mouse Genome Informatics (MGI) resource, has remained constant over the last few years. MGI has averaged a selection for curation of 12,600 papers per publication year over the last 10 years. Recent improvements to the literature mining process within the MGI system include the support for retrieval of full-text articles and semi-automated triage of journals relevant to mouse genetics and genomics research. Machine learning approaches have been deployed that select and tag publications for specific types of curatable mouse data. Our experience is that data mining approaches, combined with quality reports and expert evaluation and ultimate curation by MGI curators, results in comprehensive and consistent ability to capture relevant experimental assertions. Similar machine learning approaches will provide a general framework for us to contribute to a centralized Alliance Literature Curation Portal that will provide curators with an interface to query for all papers that have been indexed/triaged for given species, data types/methods, named entities, and in future, relevant sentences for fact extraction.
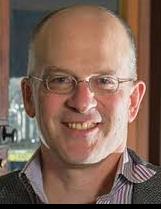
Paul Sternberg
California Institute of Technology
Biocuration: getting to the future
Abstract: I will discuss the improvements in curation efficiency implemented by WormBase as well as those planned by the Alliance of Genome Resources. WormBase started expert curation in 2000, developing web-accessible forms specific to each data type with pull-down menus and autocomplete functionality using controlled vocabularies (ontologies) and entity lists. By 2004, curators were assisted by sentence-level literature searches powered by the Textpresso Natural Language Processing (NLP) system. In 2009, we implemented semi-automatic curation, that is, presentation of NLP or regular expression text mining results for curator review. In 2014, we implemented author curation with curator review for select data types, and from 2019, NLP results are presented to authors for validation and review. Presenting NLP and other textmined results to authors is an effective combination of technology and community participation for increasing the efficiency of curation, but the extent and timing of author input is difficult to regulate. To further increase efficiency and accuracy, we are piloting curation by authors as an intrinsic part of the scholarly communication publication process, using the new journal microPublication Biology, which is controlled by biocurators. Textpresso NLP and curation is being expanded for use by other members of the Alliance of Genome Resources.
https://wormbase.org/
https://www.alliancegenome.org/
http://Textpresso.org/
https://www.micropublication.org/
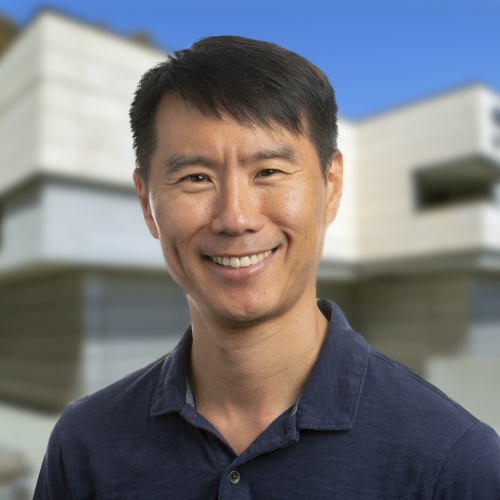
Andrew Su
Scripps Research Institute
Crowd-powered curation in Wikidata to create a FAIR biomedical knowledge graph
Wikidata is a community-maintained knowledge base that epitomizes the FAIR principles of Findability, Accessibility, Interoperability, and Reusability. Here, I will describe the breadth and depth of biomedical knowledge contained within Wikidata, assembled from primary knowledge repositories on genomics, proteomics, genetic variants, pathways, chemical compounds, and diseases. We built a collection of open-source tools that simplify the addition and synchronization of Wikidata with source databases. I will describe several use cases of how the continuously updated, crowd-contributed knowledge in Wikidata can be mined. These use cases cover a diverse cross section of biomedical analyses, from crowdsourced curation of biomedical ontologies, to phenotype-based diagnosis of disease, to drug repurposing.
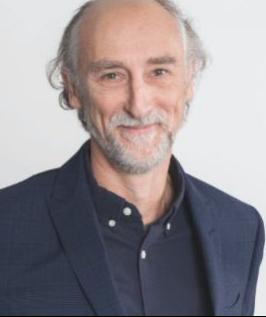
Alfonso Valencia
Barcelona Supercomputing Center
Systematic Benchmarking to Build Trust
Developers and users of Computational Biology methods are struggling with the
difficulty of assessing the
many available methods; even more now with the avalanche of ML based approaches.
A number of benchmarking
initiatives, including BioCreative
(see: https://biocreative.bioinformatics.udel.edu), are
addressing these needs in the
BioNLP domain, following a traditional schema of evaluation campaigns. These
efforts
have been quite successful in determining the quality of generated results,
monitoring progress, setting
goals and opening fields, and, importantly, providing annotated gold and silver
corpora for training and
testing. Still, they have been less
successful in creating trust and attracting users, despite the good will of
developers and users (see the
BioCreative efforts promoting text mining interfaces in the biocurators
meetings). Some of possible reasons
behind this perceive disconnection
are: challenges are designed for developers rather than for users, they are not
continuous in time, the
results are not linked to the tools that produced them. and integrating new
tools in the complex biocuration
workflows has traditionally been
technically and operationally difficult (see: https://doi.org/10.1093/database/bas020)
In the context of the European infrastructure ELIXIR, we are developing
OpenEBench - a successor of systems
such as EVA and LifeBench , as a framework for the continuous evaluation of
bioinformatics methods in
collaboration with different communities,
(so far: Multiple Sequence Alignments, Orthologs Detection, Structural Protein
predictions, and text-mining,
see https://doi.org/10.1101/181677). OpenEBench
includes the technology to host
training and testing datasets and the scoring schemas used by each community,
together with the tools for
open result exploration. In the text mining filed, OpenEBench could provide
users - and potentially curators
- with a fully accessible and
up-to-date panorama of the technologies available for a given type of problem.
https://en.wikipedia.org/wiki/Alfonso_Valencia
https://www.bsc.es/valencia-alfonso
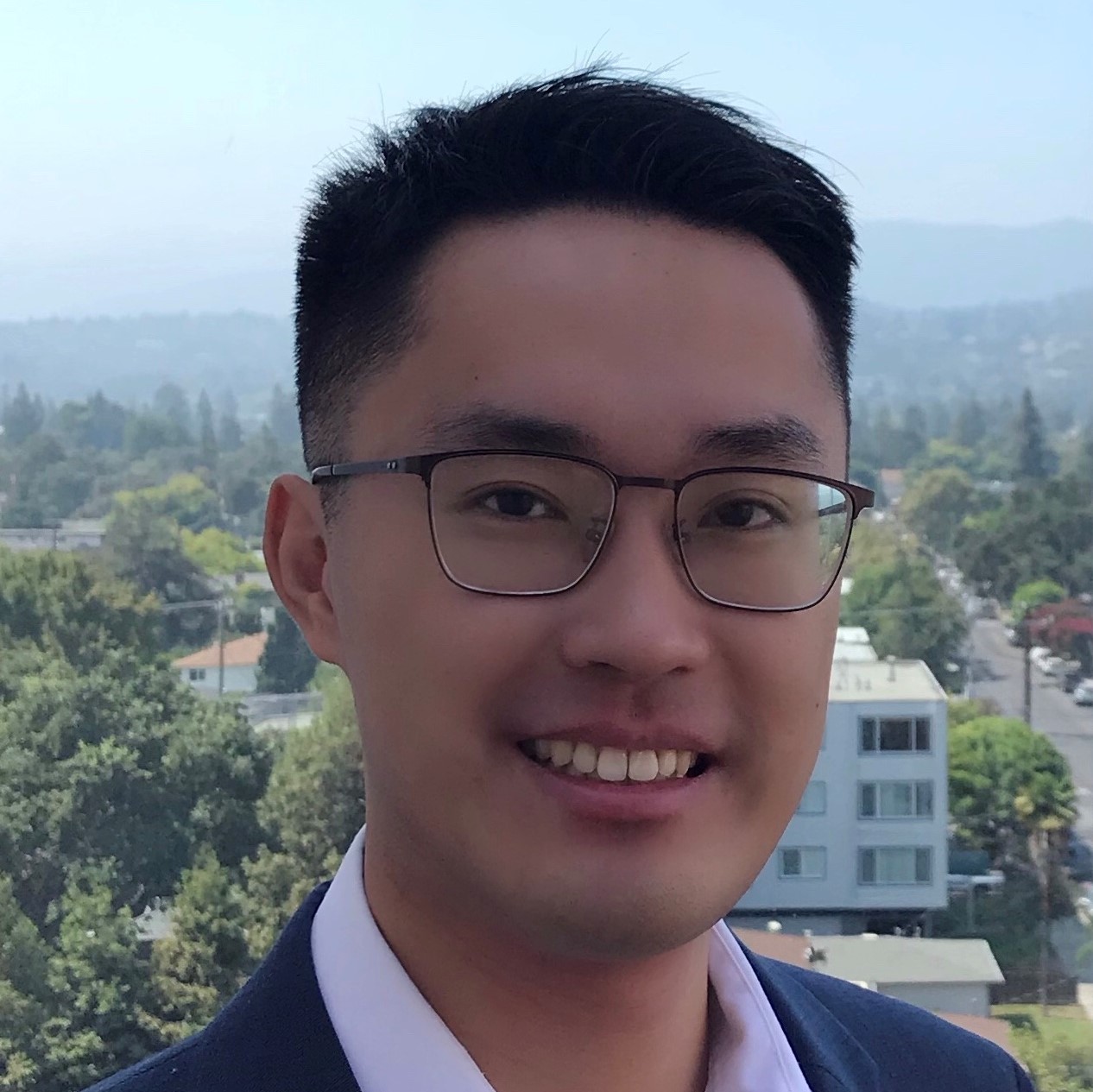
Sheng Wang
University of Washington
Precision curation: Generating sentence descriptions for new biomedical discoveries
Abstract: Current paradigm of biomedical curation relies on controlled vocabularies (CVs), where each data instance is manually or automatically curated into one term from a pre-defined set of CVs. This paradigm can effectively help us curate and analyze a small dataset, but could be limited to characterize the whole dataset since CVs cannot be used to annotate new discoveries and are not expressive enough. We propose to address the “one term fits all” problem using a new paradigm named precision curation. Each data instance in our precision curation paradigm will be curated using sentences, which is a combination of words by analogy with the drug combination therapy in precision medicine. I will present our recent efforts in generating sentences to describe terminology, pathways and protein sequences and how that leads to curation of new discoveries.
Bio: Dr. Sheng Wang is a Postdoctoral Researcher in Prof. Russ Altman's lab at Stanford. He is also a Chan Zuckerberg Biohub Scholar. His research interests lie in bionlp and computational biology. Dr. Wang has published more than twenty papers in peer review conferences and journals, including Nature Communications, Nature Machine Intelligence, and Bioinformatics.