HTA 101: II. FUNDAMENTAL CONCEPTS
- A. Health Technology
- B. Health Technology Assessment
- C. Properties and Impacts Assessed
- D. Expertise for Conducting HTA
- E. Basic HTA Frameworks
- References for Chapter II
A. Health Technology
Technology is the practical application of knowledge. Health technology is the practical application of knowledge to improve or maintain individual and population health. Three ways to describe health technology include its physical nature, its purpose, and its stage of diffusion.
1. Physical Nature
For many people, the term “technology” connotes mechanical devices or instrumentation; to others, it is a short form of “information technology,” such as computers, networking, software, and other equipment and processes to manage information. However, the practical application of knowledge in health care is quite broad. Main categories of health technology include the following.
- Drugs: e.g., aspirin, beta-blockers, antibiotics, cancer chemotherapy
- Biologics: e.g., vaccines, blood products, cellular and gene therapies
- Devices, equipment and supplies: e.g., cardiac pacemaker, magnetic resonance imaging (MRI) scanner, surgical gloves, diagnostic test kits, mosquito netting
- Medical and surgical procedures: e.g., acupuncture, nutrition counseling, psychotherapy, coronary angiography, gall bladder removal, bariatric surgery, cesarean section
- Public health programs: e.g., water purification system, immunization program, smoking prevention program
- Support systems: e.g., clinical laboratory, blood bank, electronic health record system, telemedicine systems, drug formulary,
- Organizational and managerial systems: e.g., medication adherence program, prospective payment using diagnosis-related groups, alternative health care delivery configurations
Certainly, these categories are interdependent; for example, vaccines are biologics that are used in immunization programs, and screening tests for pathogens in donated blood are used by blood banks.
2. Purpose or Application
Technologies can also be grouped according to their health care purpose, i.e.:
- Prevention: protect against disease by preventing it from occurring, reducing the risk of its occurrence, or limiting its extent or sequelae (e.g., immunization, hospital infection control program, fluoridated water supply)
- Screening: detect a disease, abnormality, or associated risk factors in asymptomatic people (e.g., Pap smear, tuberculin test, screening mammography, serum cholesterol testing)
- Diagnosis: identify the cause and nature or extent of disease in a person with clinical signs or symptoms (e.g., electrocardiogram, serological test for typhoid, x-ray for possible broken bone)
- Treatment: intended to improve or maintain health status or avoid further deterioration (e.g., antiviral therapy, coronary artery bypass graft surgery, psychotherapy)
- Rehabilitation: restore, maintain or improve a physically or mentally disabled person's function and well-being (e.g., exercise program for post-stroke patients, assistive device for severe speech impairment, incontinence aid)
- Palliation: improve the quality of life of patients, particularly for relief of pain, symptoms, discomfort, and stress of serious illness, as well as psychological, social, and spiritual problems. (Although often provided for progressive, incurable disease, palliation can be provided at any point in illness and with treatment, e.g., patient-controlled analgesia, medication for depression or insomnia, caregiver support.)
Not all technologies fall neatly into single categories. Many tests and other technologies used for diagnosis also are used for screening. (The probability that a patient who has a positive test result for a particular disease or condition truly has that disease or condition is greatly affected by whether the test was used for screening asymptomatic patients or diagnosing symptomatic patients. See discussion of “predictive value positive,” below.) Some technologies are used for diagnosis as well as treatment, e.g., coronary angiography to diagnose heart disease and to guide percutaneous coronary interventions. Implantable cardioverter defibrillators detect potentially life-threatening heart arrhythmias and deliver electrical pulses to restore normal heart rhythm. Electronic health record systems can support all of these technological purposes or applications.
Certain “hybrid” or “combination” technologies combine characteristics of drugs, devices or other major categories of technology (Goodman 1993; Lewin Group 2001; Lauritsen 2009). Among the many examples of these are: photodynamic therapy, in which drugs are laser-activated (e.g., for targeted destruction of cancer cells); local drug delivery technologies (e.g., antibiotic bone cement, drug patches, drug inhalers, implantable drug pumps, and drug-eluting coronary artery stents); spermicidal condoms; and bioartificial organs that combine natural tissues and artificial components. Examples of hybrid technologies that have complicated regulatory approval and coverage decisions are positron-emission tomography (PET, used with radiopharmaceuticals) (Coleman 1992), metered-dose inhalers (Massa 2002), and certain targeted drugs that are developed in combination with pharmacogenomic tests that are predictive of patient response to those therapies. These pharmacogenomic test-drug combinations may require clinical trials demonstrating the clinical utility of the tests as well as the safety and efficacy of the accompanying drug (US Food and Drug Administration 2007; Hudson 2011).
3. Stage of Diffusion
Technologies may be assessed at different stages of diffusion and maturity. In general, health care technologies may be described as being:
- Future: in a conceptual stage, anticipated, or in the earliest stages of development
- Experimental: undergoing bench or laboratory testing using animals or other models
- Investigational: undergoing initial clinical (i.e., in humans) evaluation for a particular condition or indication
- Established: considered by clinicians to be a standard approach to a particular condition or indication and diffused into general use
- Obsolete/outmoded/abandoned: superseded by other technologies or demonstrated to be ineffective or harmful
Often, these stages are not clearly delineated, and technologies do not necessarily mature through them in a linear fashion. A technology may be investigational for certain indications, established for others, and outmoded or abandoned for still others, such as autologous bone marrow transplantation with high-dose chemotherapy for certain types of cancers (Rettig 2007). Many technologies undergo multiple incremental innovations after their initial acceptance into general practice (Gelijns 1994; Reiser 1994). A technology that was once considered obsolete may return to established use for a better-defined or entirely different clinical purpose. A prominent example is thalidomide, whose use as a sedative during pregnancy was halted 50 years ago when it was found to induce severe fetal malformation, but which is now used to treat such conditions as leprosy, advanced multiple myeloma, chronic graft vs. host disease, and certain complications of HIV infection (Breitkreutz 2008; Zhou 2013).
B. Health Technology Assessment
Health technology assessment (HTA) is the systematic evaluation of properties, effects or other impacts of health technology. The main purpose of HTA is to inform policymaking for technology in health care, where policymaking is used in the broad sense to include decisions made at, e.g., the individual or patient level, the level of the health care provider or institution, or at the regional, national and international levels. HTA may address the direct and intended consequences of technologies as well as their indirect and unintended consequences. HTA is conducted by interdisciplinary groups using explicit analytical frameworks, drawing from a variety of methods.
1. Purposes of HTA
HTA can be used in many ways to advise or inform technology-related policies and decisions. Among these are to advise or inform:
- Regulatory agencies about whether to permit the commercial use (e.g., marketing) of a drug, device or other regulated technology
- Payers (health care authorities, health plans, drug formularies, employers, etc.) about technology coverage (whether or not to pay), coding (assigning proper codes to enable reimbursement), and reimbursement (how much to pay)
- Clinicians and patients about the appropriate use of health care interventions for a particular patient’s clinical needs and circumstances
- Health professional associations about the role of a technology in clinical protocols or practice guidelines
- Hospitals, health care networks, group purchasing organizations, and other health care organizations about decisions regarding technology acquisition and management
- Standards-setting organizations for health technology and health care delivery regarding the manufacture, performance, appropriate use, and other aspects of health care technologies
- Government health department officials about undertaking public health programs (e.g., immunization, screening, and environmental protection programs)
- Lawmakers and other political leaders about policies concerning technological innovation, research and development, regulation, payment and delivery of health care
- Health care technology companies about product development and marketing decisions
- Investors and companies concerning venture capital funding, acquisitions and divestitures, and other transactions concerning health care product and service companies
- Research agencies about evidence gaps and unmet health needs
Many of the types of organizations noted above, including government and commercial payers, hospital networks, health professional organizations, and others, have their own HTA units or functions. Many HTA agencies are affiliated with national or regional governments or consortia of multiple organizations. Further, there are independent not-for-profit and for-profit HTA organizations.
HTA contributes in many ways to the knowledge base for improving the quality of health care, especially to support development and updating of a wide spectrum of standards, guidelines, and other health care policies. For example, in the US, the Joint Commission (formerly JCAHO) and the National Committee for Quality Assurance (NCQA) set standards for measuring quality of care and services of hospitals, managed care organizations, long-term care facilities, hospices, ambulatory care centers, and other health care institutions. The National Quality Forum (NQF) endorses national evidence-based consensus standards for measuring and reporting across a broad range of health care interventions.
Health professional associations (e.g., American College of Cardiology, American College of Physicians, American College of Radiology) and special panels (e.g., the US Preventive Services Task Force, the joint Department of Veterans Affairs/Department of Defense Clinical Practice Guidelines program) develop clinical practice guidelines, standards, and other statements regarding the appropriate use of technologies (see, e.g., Institute of Medicine 2011). The Guidelines International Network (G-I-N) of organizations and individual members from more than 40 countries supports evidence-based guideline development, adaptation, dissemination, and implementation toward reducing inappropriate practice variation throughout the world. The National Guideline Clearinghouse (NGC, sponsored by the US Agency for Healthcare Research and Quality), is a searchable database of evidence-based clinical practice guidelines. Among the criteria for a new guideline to be included in NGC effective June 2014 is that it be based on a carefully documented systematic review of the evidence, including a detailed search strategy and description of study selection.
Standards-setting organizations such as the American National Standards Institute (ANSI) and the American Society for Testing and Materials coordinate development of voluntary national consensus standards for the manufacture, use, and reuse of health devices and their materials and components. For example, ANSI has developed standards and specifications for electronic information sharing and interoperability in such areas as laboratory results reporting, medication management, personalized health care, immunizations, and neonatal screening (Kuperman 2010).
As noted above, HTA can be used to support decision making by clinicians and patients. The term evidence-based medicine refers to the use of current best evidence from scientific and medical research, and the application of clinical experience and observation, in making decisions about the care of individual patients (Glasziou 2011; Straus 2011). This prompted the appearance of many useful resources, including:
- Evidence-Based Medicine (Sackett 1997), a guide to the field, recently updated (Straus 2011)
- Evidence-Based Medicine (a joint product of the American College of Physicians and the BMJ Publishing Group), a journal digest of articles selected from international medical journals
- “Users’ guides to the medical literature,” a series of more than 30 articles by the Evidence-Based Medicine Working Group, originally published in the Journal of the American Medical Association, starting in the 1990s and more recently assembled and updated (Guyatt 2008)
- Centre for Evidence-Based Medicine
2. Basic HTA Orientations
The impetus for an HTA is not necessarily a particular technology. Three basic orientations to HTA are as follows.
- Technology-oriented assessments are intended to determine the characteristics or impacts of particular technologies. For example, a government agency may want to determine the clinical, economic, social, professional, or other impacts of cochlear implants, cervical cancer screening, PET scanners, or widespread adoption of electronic health record systems.
- Problem-oriented assessments focus on solutions or strategies for managing a particular disease, condition, or other problem for which alternative or complementary technologies might be used. For example, clinicians and other providers concerned with the problem of diagnosis of dementia may call for HTA to inform the development of clinical practice guidelines involving some combination or sequence of clinical history, neurological examination, and diagnostic imaging using various modalities.
- Project-oriented assessments focus on a local placement or use of a technology in a particular institution, program, or other designated project. For example, this may arise when a hospital must decide whether or not to purchase a PET scanner, considering the facilities, personnel, and other resources needed to install and operate a PET scanner; the hospital’s financial status; local market potential for PET services; competitive factors; etc.
These basic assessment orientations can overlap and complement one another. Certainly, all three types could draw on a common body of scientific evidence and other information. A technology-oriented assessment may address the range of problems for which the technology might be used and how appropriate the technology might be for different types of local settings (e.g., inpatient versus outpatient). A problem-oriented assessment may compare the effectiveness, safety, and other impacts of alternative technologies for a given problem, e.g., alternative treatments for atrial fibrillation (e.g., drug therapy, surgery, or catheter ablation), and may draw on technology-oriented assessments of one or more of those alternatives as well as any direct (“head-to-head”) comparisons of them. A project-oriented assessment would consider the range of impacts of a technology or its alternatives in a given setting, as well as the role or usefulness of that technology for various problems. Although the information used in a project-oriented assessment by a particular hospital may include findings of pertinent technology- and problem-oriented assessments, local data collection and analysis may be required to determine what is appropriate for that hospital. Thus, many HTAs will blend aspects of all three basic orientations.
C. Properties and Impacts Assessed
What does HTA assess? HTA may involve the investigation of one or more properties, impacts, or other attributes of health technologies or applications. In general, these include the following.
- Technical properties
- Safety
- Efficacy and/or effectiveness
- Economic attributes or impacts
- Social, legal, ethical and/or political impacts
The properties, impacts, and other attributes assessed in HTA pertain across the range of types of technology. Thus, for example, just as drugs, devices, and surgical procedures can be assessed for safety, effectiveness, and cost effectiveness, so can hospital infection control programs, computer-based drug-utilization review systems, and rural telemedicine networks.
Technical properties include performance characteristics and conformity with specifications for design, composition, manufacturing, tolerances, reliability, ease of use, maintenance, etc.
Safety is a judgment of the acceptability of risk (a measure of the probability of an adverse outcome and its severity) associated with using a technology in a given situation, e.g., for a patient with a particular health problem, by a clinician with certain training, or in a specified treatment setting.
Efficacy and effectiveness both refer to how well a technology works, i.e., accomplishes its intended purpose, usually based on changes in one or more specified health outcomes or “endpoints” as described below. A technology that works under carefully managed conditions does not always work as well under more heterogeneous or less controlled conditions. In HTA, efficacy refers to the benefit of using a technology for a particular problem under ideal conditions, e.g., within the protocol of a carefully managed RCT, involving patients meeting narrowly defined criteria, or conducted at a “center of excellence.” Effectiveness refers to the benefit of using a technology for a particular problem under general or routine conditions, e.g., by a physician in a community hospital for a variety of types of patients. Whereas efficacy answers the question, “Can it work?” (in the best conditions), effectiveness answers the question “Does it work?” (in real-world conditions).
Clinicians, patients, managers and policymakers are increasingly aware of the practical implications of differences in efficacy and effectiveness. Researchers delve into registers, databases (e.g., of third-party payment claims and administrative data), and other epidemiological and observational data to discern possible associations between the use of technologies and patient outcomes in general or routine practice settings. As these are observational studies, their validity for establishing causal connections between interventions and patient outcomes is limited compared to experimental studies, particularly RCTs. Even so, observational studies can be used to generate hypotheses for experimental trials, and they can provide evidence about effectiveness that can complement other evidence about efficacy, suggesting whether findings under ideal conditions may be extended to routine practice. As discussed below, some different types of trials are designed to incorporate varied groups of patients and settings.
Box II-1 shows certain distinctions in efficacy and effectiveness for diagnostic tests. Whereas the relationship between a preventive, therapeutic, or rehabilitative technology and patient outcomes is often direct (though not always easy to measure), the relationship between a technology used for diagnosis or screening and patient outcomes is usually indirect. Also, diagnostic and screening procedures can have their own short-term and long-term adverse health effects, e.g., arising from biopsies, certain radiological procedures, or genetic testing for certain disorders.
Box II-1. Efficacy vs. Effectiveness for Diagnostic Tests
Efficacy | Effectiveness | |
---|---|---|
Patient Population | Homogeneous; patients with coexisting illness often excluded | Heterogeneous; includes all patients who usually have test |
Procedures | Standardized | Often variable |
Testing Conditions | Ideal | Conditions of everyday practice |
Practitioner | Experts | All users |
Adapted from: Institute of Medicine 1989.
Economic attributes or impacts of health technologies can be microeconomic and macroeconomic. Microeconomic concerns include costs, prices, charges, and payment levels associated with individual technologies. Other concerns include comparisons of resource requirements and outcomes (or benefits) of technologies for particular applications, such as cost effectiveness, cost utility, and cost benefit. (Methods for determining these are described in chapter V, Economic Analysis Methods.) Health technology can have or contribute to a broad range of macroeconomic impacts. These include impacts on: a nation’s gross domestic product, national health care costs, and resource allocation across health care and other industrial sectors, and international trade. Health technology can also be a factor in national and global patterns of investment, innovation, competitiveness, technology transfer, and employment (e.g., workforce size and mobility). Other macroeconomic issues that pertain to health technologies include the effects of intellectual property policies (e.g., for patent protection), regulation, third-party payment, and other policy changes that affect technological innovation, adoption, diffusion, and use.
Ethical, legal, and social considerations arise in HTA in the form of normative concepts (e.g., valuation of human life); choices about how and when to use technologies; research and the advancement of knowledge; resource allocation; and the integrity of HTA processes themselves (Heitman 1998). Indeed, the origins of technology assessment called for the field to support policymakers’ broader considerations of technological impacts, such as the “social, economic, and legal implications of any course of action” (US Congress, House of Representatives 1967) and the “short- and long-term social consequences (for example, societal, economic, ethical, legal) of the application of technology” (Banta 1993). More recently, for example, an integral component of the Human Genome Project of the US National Institutes of Health is the Ethical, Legal and Social Implications (ELSI) Research Program (Green 2011). One recently proposed broader framework, “HELPCESS,” includes consideration of: humanitarian, ethical, legal, public relationships, cultural, economic, safety/security, and social implications (Yang 2013).
Whether in health care or other sectors, technological innovation can challenge certain ethical, religious, cultural, and legal norms. Current examples include genetic testing, use of stem cells to grow new tissues, allocation of scarce organs for transplantation, and life-support systems for critically ill patients. For example, the slowly increasing supply of donated kidneys, livers, hearts, lungs, and other solid organs for transplantation continues to fall behind the expanding need for them, raising ethical, social, and political concerns about allocation of scarce, life-saving resources (Huesch 2012; Yoshida 1998). In dialysis and transplantation for patients with end-stage renal disease, ethical concerns arise from patient selection criteria, termination of treatment, and managing non-compliant and other problem patients (Moss 2011; Rettig 1991). Even so, these concerns continue to prompt innovations to overcome organ shortages (Lechler 2005), such as techniques for improving transplantation success rates with organs from marginal donors, organs from living donors, paired and longer chain donation, xenotransplantation (e.g., from pigs), stem cells to regenerate damaged tissues, and the longer-range goal of whole-organ tissue engineering (Soto-Gutierrez 2012).
Technologies that can diminish or strengthen patient dignity or autonomy include, e.g., end-of-life care, cancer chemotherapy, feeding devices, and assistive equipment for moving immobilized patients. Greater involvement of patients, citizens, and other stakeholders in health care decisions, technology design and development, and the HTA process itself is helping to address some concerns about the relationships between patients and health technology. Ethical questions also have led to improvements in informed consent procedures for patients involved in clinical trials.
Allocation of scarce resources to technologies that are expensive, misused, not uniformly accessible, or non-curative can raise broad concerns about equity and squandered opportunities to improve population health (Gibson 2002). The same technologies can pose various challenges in the context of different or evolving societal and cultural norms, economic conditions, and health care system delivery and financing configurations. Even old or “mainstream” technologies can raise concerns in changing social contexts, such as immunization, organ procurement for transplantation, or male circumcision (EUnetHTA 2008). In addition to technologies, certain actual or proposed uses of analytical methods can prompt such concerns; many observers object to using actual or implied cost per quality-adjusted life year (QALY) thresholds in coverage decisions (Nord 2010).
Methods for assessing ethical, legal, and social implications of health technology have been underdeveloped relative to other methods in HTA, although there has been increased attention in recent years to developing frameworks and other guidance for these analyses (Duthie 2011; Potter 2008). More work is needed for translating these implications into policy (Van der Wilt 2000), such as for involving different perspectives in the HTA process in order to better account for identification of the types of effects or impacts that should be assessed, and for values assigned by these different perspectives to life, quality of life, privacy, choice of care, and other matters (Reuzel 2001). Some methods used in analysis of ethical issues in HTA, based on work assembled by the European network for Health Technology Assessment (EUnetHTA), are listed in Box II-2. Recent examination of alternative methods used in ethical analysis in HTA suggests that they can yield similar results, and that having a systematic and transparent approach to ethical analysis is more important than the choice of methods (Saarni 2011).
Box II-2. Methods Used for Ethical Analysis in HTA
Method | Description |
Casuistry | Solves morally challenging situations by comparing them with relevant and similar cases where an undisputed solution exists |
Coherence analysis | Tests the consistency of ethical argumentation, values or theories on different levels, with an ideal goal of a logically coherent set of arguments |
Principlism | Approaches ethical problems by addressing basic ethical principles, rooted in society’s common morality |
Interactive, participatory HTA approaches | Involves different stakeholders in a real discourse, to reduce bias and improve the validity and applicability of the HTA |
Social shaping of technology | Addresses the interaction between society and technology and emphasizes how to shape technology in the best ways to benefit people |
Wide reflective equilibrium | Aims at a coherent conclusion by a process of reflective mutual adjustment among general principles and particular judgements |
Source: Saarni et al. 2008.
As a form of objective scientific and social inquiry, HTA must be subject to ethical conduct, social responsibility, and cultural differences. Some aspects to be incorporated or otherwise addressed include: identifying and minimizing potential conflicts of interest on the part of assessment staff and expert advisors; accounting for social, demographic, economic, and other dimensions of representativeness and equity in HTA resource allocation and topic selection; and patient and other stakeholder input on topic selection, evidence questions, and relevant outcomes/endpoints.
The terms “appropriate” and “necessary” often are used to describe whether or not a technology should be used in particular circumstances. These are judgments that typically reflect considerations of one or more of the properties and impacts described above. For example, the appropriateness of a diagnostic test may depend on its safety and effectiveness compared to alternative available interventions for particular patient indications, clinical settings, and resource constraints, perhaps as summarized in an evidence-based clinical practice guideline. A technology may be considered necessary if it is likely to be effective and acceptably safe for particular patient indications, and if withholding it would be deleterious to the patient's health (Hilborne 1991; Kahan 1994; Singer 2001).
As described in chapter I, HTA inquires about the unintended consequences of health technologies as well an intended ones, which may involve some or all of the types of impacts assessed. Some unintended consequences include, or lead to, unanticipated uses of technologies. Box II-3 lists some recent examples.
Box II-3. Recent Examples of Unintended Consequences of Health Technology
Technology | Intended or Original Uses | Unintended Consequences or Unanticipated Uses |
---|---|---|
Antibiotics (antibacterials) | Kill or inhibit growth of bacteria that cause infectious diseases | Overuse and improper use leading to multi-drug resistant bacterial strains1 |
Antiretroviral therapy (ART) | Treatment of HIV/AIDS | Return to risky sexual behaviors in some patient groups2,3,4 |
Aspirin | Relieve pain, fever, inflammation | Antiplatelet to prevent blood clots5 |
Bariatric surgery | Weight loss in obese patients | Cure or remission of type 2 diabetes in many of the obese patients6 |
Medical ultrasonography | Visualizing structures and blood flow in the body in real time | Fetal sex selection7,8,9 |
Prostate cancer screening with PSA test | Identify men with prostate cancer early enough to cure | Invasive testing, therapies, and adverse effects for men with slow-growing/low-risk cases that will never cause symptoms10,11 |
Sildenafil | Cardiovascular disorders, especially hypertension (used today for pulmonary arterial hypertension) | Treat male sexual dysfunction12 |
Sources:
1Hollis A, Ahmed Z. Preserving antibiotics, rationally. N Engl J Med. 2013;369(26):2474-6.
2Fu TC, et al. Changes in sexual and drug-related risk behavior following antiretroviral therapy initiation among HIV-infected injection drug users. AIDS. 2012;26(18):2383-91.
3Kembabazi A, et al. Disinhibition in risky sexual behavior in men, but not women, during four years of antiretroviral therapy in rural, southwestern Uganda. PLoS One. 2013;8(7):e69634.
4Tun W, et al. Increase in sexual risk behavior associated with immunologic response to highly active antiretroviral therapy among HIV-infected injection drug users. Clin Infect Dis. 2004;38(8):1167-74.
5Hackam DG, Eikelboom JW. Antithrombotic treatment for peripheral arterial disease. Heart. 2007;93(3):303-8.
6Brethauer SA, et al. Can diabetes be surgically cured? Long-term metabolic effects of bariatric surgery in obese patients with type 2 diabetes mellitus. Ann Surg. 2013;258(4):628-36.
7George SM. Millions of missing girls: from fetal sexing to high technology sex selection in India. Prenat Diagn. 2006 Jul;26(7):604-9.
8Nie JB. Non-medical sex-selective abortion in China: ethical and public policy issues in the context of 40 million missing females. Br Med Bull. 2011;98:7-20.
9Thiele AT, Leier B. Towards an ethical policy for the prevention of fetal sex selection in Canada. J Obstet Gynaecol Can. 2010 Jan;32(1):54-7.
10Hayes JH, Barry MJ. Screening for prostate cancer with the prostate-specific antigen test: a review of current evidence. JAMA. 2014;311(11):1143-9.
11Lin K, Lipsitz R, Miller T, Janakiraman S; U.S. Preventive Services Task Force. Benefits and harms of prostate-specific antigen screening for prostate cancer: an evidence update for the U.S. Preventive Services Task Force. Ann Intern Med. 2008;149(3):192-9.
12Kling J. From hypertension to angina to Viagra. Mod Drug Discov. 1998;1(2):31-8.
1. Measuring Health Outcomes
Health outcome variables are used to measure the safety, efficacy and effectiveness of health care technologies. Main categories of health outcomes are:
- Mortality (death rate)
- Morbidity (disease rate)
- Adverse health events (e.g., harmful side effects)
- Quality of life
- Functional status
- Patient satisfaction
For example, for a cancer treatment, the main outcome of interest may be five-year survival rate; for treatments of coronary artery disease, the main endpoints may be incidence of fatal and nonfatal acute myocardial infarction (heart attack) and recurrence of angina pectoris (chest pain due to poor oxygen supply to the heart). Although mortality, morbidity, and adverse events are usually the outcomes of greatest interest, the other types of outcomes are often important as well to patients and others. Many technologies affect patients, family members, providers, employers, and other interested parties in other important ways; this is particularly true for many chronic diseases. As such, there is increasing emphasis on quality of life, functional status, patient satisfaction, and related types of patient outcomes.
In a clinical trial and other studies comparing alternative treatments, the effect on health outcomes of one treatment relative to another (e.g., a new treatment vs. a control treatment) can be expressed using various measures of treatment effect. These measures compare the probability of a given health outcome in the treatment group with the probability of the same outcome in a control group. Examples are absolute risk reduction, odds ratio, number needed to treat, and effect size. Box II-4 shows how choice of treatment effect measures can give different impressions of study results.
Box II-4. Choice of Treatment Effect Measures Can Give Different Impressions
A study of the effect of breast cancer screening can be used to contrast several treatment effect measures and show how they can give different impressions about the effectiveness of an intervention (Forrow 1992). In 1988, Andersson (1988) reported the results of a large RCT that was conducted to determine the effect of mammographic screening on mortality from breast cancer. The trial involved more than 42,000 women who were over 45 years old. Half of the women were invited to have mammographic screening and were treated as needed. The other women (control group) were not invited for screening.
The report of this trial states that "Overall, women in the study group aged >55 had a 20% reduction in mortality from breast cancer." Although this statement of relative risk reduction is true, it is based on the reduction from an already low-probability event in the control group to an even lower one in the screened group. Calculation of other types of treatment effect measures provides important additional information. The table below shows the number of women aged 55 and breast cancer deaths in the screened group and control group, respectively. Based on these results, four treatment effect measures are calculated.
For example, absolute risk reduction is the difference in the rate of adverse events between the screened group and the control group. In this trial, the absolute risk reduction of 0.0007 means that the absolute effect of screening was to reduce the incidence of breast cancer mortality by 7 deaths per 10,000 women screened, or 0.07%.
Group | No. of Patients | Deaths from breast cancer | Probability of death from breast cancer | Relative risk reduction1 | Absolute reduction2 | Odds ratio3 | No. needed to screen4 |
---|---|---|---|---|---|---|---|
Screened | 13,107 | 35 | Pc= 0.0027 | 20.6% | 0.0007 | 0.79 | 1,429 |
Control | 13,113 | 44 | Pc= 0.0034 | ─ | ─ | ─ | ─ |
Women in the intervention group were invited to attend mammographic screening at intervals of 18-24 months. Five rounds of screening were completed. Breast cancer was treated according to stage at diagnosis. Mean follow-up was 8.8 years.
1. Relative risk reduction: (Pc- Ps) ÷ Pc
2. Absolute risk reduction: Pc- Ps
3. Odds ratio: [Ps÷ (1 - Ps)] ÷ [Pc÷ (1 - Pc)]
4. Number needed to screen to prevent one breast cancer death: 1 ÷ (Pc- Ps)
Source of number of patients and deaths from breast cancer: Andersson 1988
a. Biomarkers and Surrogate Endpoints
Certain health outcomes or clinical endpoints have particular roles in clinical trials, other research, and HTA, including biomarkers, intermediate endpoints, and surrogate endpoints.
A biomarker (or biological marker) is an objectively measured variable or trait that is used as an indicator of a normal biological process, a disease state, or effect of a treatment (Biomarkers Definitions Working Group 2001). It may be a physiological measurement (height, weight, blood pressure, etc.), blood component or other biochemical assay (red blood cell count, viral load, glycated hemoglobin [HbA1c] level, etc.), genetic data (presence of a specific genetic mutation), or measurement from an image (coronary artery stenosis, cancer metastases).
An intermediate endpoint is a non-ultimate endpoint (e.g., not mortality or morbidity) that may be associated with disease status or progression toward an ultimate endpoint such as mortality or morbidity. They include certain biomarkers (e.g., HbA1c in prediabetes or diabetes, bone density in osteoporosis, tumor progression in cancer) or disease symptoms (e.g., angina frequency in heart disease, measures of lung function in chronic obstructive pulmonary disease). Some intermediate endpoints can serve as surrogate endpoints.
A surrogate endpoint is a measure (typically a biomarker) that is used as a substitute for a clinical endpoint of interest, such as morbidity and mortality. They are used in clinical trials when it is impractical to measure the primary endpoint during the course of the trial, such as when observation of the clinical endpoint would require years of follow-up. A surrogate endpoint is assumed, based on scientific evidence, to be a valid and reliable predictor of a clinical endpoint of interest. As such, changes in a surrogate endpoint should be highly correlated with changes in the clinical endpoint. For example, a long-standing surrogate marker for risk of stroke is hypertension, although understanding continues to evolve of the respective and joint roles of systolic and diastolic pressures in predicting stroke in the general population and in high-risk populations (Malyszko 2013). RCTs of new drugs for HIV/AIDS use biological markers such as virological (e.g., plasma HIV RNA) levels (or “loads”) and immunological (e.g., CD4+ cell counts) levels (Lalezari 2003) as surrogates for mortality and morbidity. Other examples of surrogate endpoints for clinical endpoints are negative cultures for cures of bacterial infections and decrease of intraocular pressure for loss of vision in glaucoma.
b. Quality of Life Measures
Quality of life (QoL) measures, or “health-related quality of life” measures or indexes, are increasingly used along with more traditional outcome measures to assess efficacy and effectiveness, providing a more complete picture of the ways in which health care affects patients. QoL measures capture such dimensions (or domains) as: physical function, social function, cognitive function, anxiety/distress, bodily pain, sleep/rest, energy/fatigue and general health perception. These measures may be generic (covering overall health) or disease-specific. They may provide a single aggregate score or yield a set of scores, each for a particular dimension. Some examples of widely used generic measures are:
- CAHPS (formerly Consumer Assessment of Healthcare Providers and Systems)
- EuroQol (EQ-5D)
- Health Utilities Index
- Nottingham Health Profile
- Quality of Well-Being Scale
- Short Form (12) Health Survey (SF-12)
- Short Form (36) Health Survey (SF-36)
- Sickness Impact Profile
Dimensions of selected generic QoL measures that have been used extensively and that are well validated for certain applications are shown in Box II-5. There is an expanding literature on the relative strengths and weaknesses of these generic QoL indexes, including how sensitive they are to changes in quality of life for people with particular diseases and disorders (Coons 2000; Feeny 2011; Fryback 2007; Kaplan 2011; Kaplan 1998; Post 2001; Saban 2008).
Box II-5. Domains of Selected General Health-Related Quality of Life Indexes
EuroQol EQ-5D (Rabin 2001)
· Mobility |
· Pain/discomfort |
· Self-care |
· Anxiety/depression |
· Usual activities |
Functional Independence Measure (Hsueh 2002; Linacre 1994)
· Self-care |
· Communication |
· Sphincter control |
· Psychosocial |
· Mobility |
· Cognition |
Nottingham Health Profile (Doll 1993; Jenkinson 1988)
· Physical mobility |
· Energy |
· Pain |
· Social isolation |
· Sleep |
· Emotional reactions |
Quality of Well-Being Scale (Frosch 2004; Kaplan 1989)
· Mobility |
· Social activity |
· Physical activity |
· Symptom-problem complex |
Short Form (SF)-36 (Martin 2011; Ware 1992)
· Physical functioning |
· Mental health |
· Role - physical |
· Role - emotional |
· Social functioning |
· Vitality |
· Bodily pain |
· General health perceptions |
Sickness Impact Profile (Bergner 1981; de Bruin 1992)
· Body care and movement |
· Emotional behavior |
· Ambulation |
· Alertness behavior |
· Mobility |
· Communication |
· Sleep and rest |
· Social interaction |
· Home management |
· Work |
· Recreation and pastimes |
· Eating |
Some of the diseases or conditions for which there are disease- (or condition-) specific measures are: angina, arthritis, asthma, epilepsy, heart disease, kidney disease, migraine, multiple sclerosis, urinary incontinence, and vision problems. See Box II-6 for dimensions used in selected measures.
Box II-6. Domains of Selected Disease-Specific Health-Related Quality of Life Indexes
Adult Asthma Quality of Life Questionnaire (Juniper 2005; Juniper 1993)
· Activity limitations |
· Exposure to environmental stimuli |
· Emotional function |
· Symptoms |
Arthritis Impact Measurement Scales (AIMS2) (Söderlin 2004; Meenan 1992)
· Mobility |
· Social activity |
· Walking and bending |
· Support from family and friends |
· Hand and finger function |
· Arthritis pain |
· Arm function |
· Work |
· Self care |
· Level of tension |
· Household tasks |
· Mood |
Urinary Incontinence-Specific Quality of Life Instrument (I-QOL) (Patrick 1999; Wagner 1996)
· Avoidance and limiting behavior |
· Social embarrassment |
· Psychosocial impacts |
Considerable advances have been made in the development and validation of generic and disease-specific measures since the 1980s. These measures are increasingly used by health product companies to differentiate their products from those of competitors, which may have virtually indistinguishable effects on morbidity for particular diseases (e.g., hypertension, depression, arthritis) but may have different side effect profiles that affect patients’ quality of life (Gregorian 2003).
c. Health-Adjusted Life Years: QALYs, DALYs, and More
The category of measures known as health-adjusted life years (HALYs) recognizes that changes in an individual’s health status or the burden of population health should reflect not only the dimension of life expectancy but a dimension of QoL or functional status. Three main types of HALYs are: quality-adjusted life years (QALYs), disability-adjusted life years (DALYs), and healthy-years equivalents (HYEs). One of the attributes of HALYs is that they are not specific to a particular disease or condition.
The QALY is a unit of health care outcome that combines gains (or losses) in length of life with quality of life. QALYs are usually used to represent years of life subsequent to a health care intervention that are weighted or adjusted for the quality of life experienced by the patient during those years (Torrance 1989). QALYs provide a common unit for multiple purposes, including: estimating the overall burden of disease; comparing the relative impact on personal and population health of specific diseases or conditions, comparing the relative impact on personal and population health of specific technologies; and making economic comparisons, such as of the cost-effectiveness (in particular the cost-utility) of different health care interventions. Some health economists and policymakers have proposed setting priorities among alternative health care interventions by selecting among these so as to maximize the additional health gain in terms of QALYs. This is intended to optimize allocation of scarce resources and thereby maximize social welfare (Gold 2002; Johannesson 1993; Mullahy 2001). QALYs are used routinely in assessing the impact or value of technologies by some HTA organizations, e.g., the National Institute for Health and Care Excellence (NICE) in the UK. Box II-7 illustrates the dual dimensions of QALYs, and how an intervention can result in a gain in QALYs.
Box II-7. Gain in Quality-Adjusted Life Years from a New Intervention
QALY = Length of life X Quality Weight
Survival and Quality of Life with Current Treatment
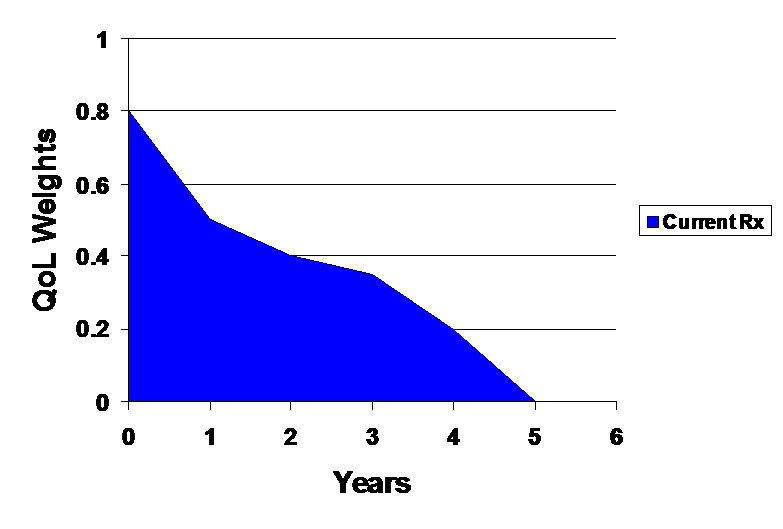
Survival and Quality of Life with New Treatment
QALY Gain is Represented by the Area of Increased Survival and Quality of Life

Although HALYs arise from a common concept of adjusting duration of life by individuals’ experience of quality of life, they differ in ways that have implications for their appropriate use, including for assessing cost-effectiveness. QALYs are used primarily to adjust a person’s life expectancy by the levels of health-related quality of life that the person is predicted to experience during the remainder of life or some interval of it. DALYs are primarily used to measure population disease burden; they are a measure of something ‘lost’ rather than something ‘gained.’ The health-related quality of life weights used for QALYs are intended to represent quality of life levels experienced by individuals in particular health states, whereas the disability weights used for DALYs represent levels of loss of functioning caused by mental or physical disability caused by disease or injury. Another key distinction is that the burden of disability in calculating DALYs depends on one’s age. That is, DALYs incorporate an age-weighting function that assigns different weights to life years lived at different ages. Also, the origins of quality of life weights and disability weights are different (Sassi 2006; Fox-Rushby 2001).
The scale of quality of life used for QALYs can be based on general, multi-attribute QoL indexes or preference survey methods (Bleichrodt 1997; Doctor 2010; Weinstein 2010). The multi-attribute QoL indexes used for this purpose include, e.g., the SF-6D (based on the SF-36), EQ-5D, versions of the Health Utilities Index, and Quality of Well-Being Scale. The preference survey methods are used to elicit the utility or preferences of individuals (including patients, disabled persons, or others) for certain states of health or well-being, such as the standard gamble, time-tradeoff, or rating scale methods (e.g., a visual analog scale). Another preference survey method, the person trade-off, is used for eliciting preferences for the health states of a community or population, although the standard gamble, time tradeoff, and rating scales can be used at that level as well. This scale is typically standardized to a range of 0.0 (death) to 1.0 (perfect health). A scale may allow for ratings below 0.0 for states of disability and distress that some patients consider to be worse than death (Patrick 1994). Some work has been done to capture more dimensions of public preference and to better account for the value attributed to different health care interventions (Dolan 2001; Schwappach 2002). There is general agreement about the usefulness of the standard measures of health outcomes such as QALYs to enable comparisons of the impacts of technologies across diseases and populations, and standard approaches for valuing utilities for different health states. Among the areas of controversy are:
- whether the QALY captures the full range of health benefits,
- that the QALY does not account for social concerns for equity
- whether the QALY is the most appropriate generic preference-based measure of utility
- whether a QALY is the same regardless of who experiences it
- what the appropriate perspective is for valuing health states, e.g., from the perspective of patients with particular diseases or the general public (Whitehead 2010).
Regarding perspective, for example, the values of the general public may not account for adaptation of the patients to changes in health states, and patients’ values may incorporate self-interest. Given this divergence, the appropriate perspective for health state valuations should depend on the context of the decisions or policies to be informed by the evaluation (Stamuli 2011; Oldridge 2008).
QoL measures and QALYs continue to be used in HTA while substantial work continues in reviewing, refining and validating them. As described in chapter V, Economic Analysis Methods, the QALY is often used as the unit of patient outcomes in cost-utility analyses.
2. Performance of Screening and Diagnostic Technologies
Screening and diagnostic tests provide information about the presence of a disease or other health condition. As such, they must be able to discriminate between patients who have a particular disease or condition and those who do not have it. Although the tests used for them are often the same, screening and diagnosis are distinct applications: screening is conducted in asymptomatic patients; diagnosis is conducted in symptomatic patients. As described below, whether a particular test is used for screening or it is used for diagnosis can have a great effect on the probability that the test result truly indicates whether or not a patient has a given disease or other health condition. Although these tests are most often recognized as being used for screening and diagnosis, there are other, related uses of these tests across the spectrum of managing a disease or condition, as listed in Box II-8.
Box II-8. Uses of Tests for Asymptomatic and Symptomatic Patients
Asymptomatic patients (no known disease)
- Susceptibility: presence of a risk factor for a disease (e.g., a gene for a particular form of cancer)
- Presence of (hidden or occult) disease (e.g., Pap smear for cervical cancer)
Symptomatic patients (known or probable disease)
- Diagnosis: presence of a particular disease or condition (e.g., thyroid tests for suspected hyperthyroidism)
- Differential diagnosis: determine which disease or condition a patient has from among multiple possible alternatives (e.g., in a process of elimination using a series of tests to rule out particular diseases or conditions)
- Staging: extent or progression of a disease (e.g., imaging to determine stages of cancer)
- Prognosis: probability of progression of a disease or condition to a particular health outcome
(e.g., a multi-gene test for survival of a particular type of cancer) - Prediction: probability of a treatment to result in progression of a disease or condition to a particular health outcome (e.g., a genetic test for the responsiveness of colorectal cancer to a particular chemotherapy)
- Surveillance: periodic testing for recurrence or other change in disease or condition status
- Monitoring: response to treatment (e.g., response to anticoagulation therapy)
The technical performance of a test depends on multiple factors. Among these are the precision and accuracy of the test, the observer variation in reading the test data, and the relationship between the disease of interest and the designated cutoff level (threshold) of the variable (usually a biomarker) used to determine the presence or absence of that disease. These factors contribute to the ability of a test to detect a disease when it is present and to not detect a disease when it is not present.
A screening or diagnostic test can have four basic types of outcomes, as shown in Box II-9. A true positive test result is one that detects a marker when the disease is present. A true negative test result is one that does not detect the marker when the disease is absent. A false positive test result is one that detects a marker when the disease is absent. A false negative test result is one that does not detect a marker when the disease is present.
Box II-9. Possible Outcomes of a Screening or Diagnostic Test
Test Result |
True Disease Status |
|
Present |
Absent |
|
Positive (+) |
True + |
False + |
Negative (-) |
False - |
True - |
Operating characteristics of tests and procedures are measures of their technical performance. These characteristics are based on the probabilities of the four possible types of outcomes of a test noted above. The two most commonly used operating characteristics of screening and diagnostic tests are sensitivity and specificity. Sensitivity measures the ability of a test to detect a particular disease (e.g., a particular type of infection) or condition (a particular genotype) when it is present. Specificity measures the ability of a test to correctly exclude that disease or condition in a person who truly does not have that disease or condition. The sensitivity and specificity of a test are independent of the true prevalence of the disease or condition in the population being tested.
A graphical way of depicting these operating characteristics for a given diagnostic test is with a receiver operating characteristic (ROC) curve, which plots the relationship between the true positive ratio (sensitivity) and false positive ratio (1 - specificity) for all cutoff points of a disease or condition marker. For a perfect test, the area under the ROC curve would be 1.0; for a useless test (no better than a coin flip), the area under the ROC curve would be 0.5. ROC curves help to demonstrate how raising or lowering a cutoff point selected for defining a positive test result affects tradeoffs between correctly identifying people with a disease (true positives) and incorrectly labeling a person as positive who does not have the disease (false positives).
Sensitivity and specificity do not reveal the probability that a given patient really has a disease if the test is positive, or the probability that a given patient does not have the disease if the test is negative. These probabilities are captured by two other operating characteristics, shown in Box II-10. Positive predictive value is the proportion of those patients with a positive test result who actually have the disease. Negative predictive value is the proportion of patients with a negative test result who actually do not have the disease. Unlike sensitivity and specificity, the positive and negative predictive values of a test do depend on the true prevalence of the disease or condition in the population being tested. That is, the positive and negative predictive values of a test result are not constant performance characteristics of a test; they vary with the prevalence of the disease or condition in the population of interest. For example, if a disease is very rare in the population, even tests with high sensitivity and high specificity can have low predictive value positive, generating more false-positive than false negative results.
Box II-10. Operating Characteristics of Diagnostic Tests
Characteristic |
Formula |
Definition |
Sensitivity |
True Positives True positives + False negatives |
Proportion of people with condition who test positive |
Specificity |
True Negatives True negatives + False positives |
Proportion of people without condition who test negative |
Positive predictive value |
True Positives True positives + False positives |
Proportion of people with positive test who have condition |
Negative predictive value |
True Negatives True negatives + False negatives |
Proportion of people with negative test who do not have condition |
a. Biomarkers and Cutoff Points in Disease Detection
The biomarker for certain diseases or conditions is typically defined as a certain cutoff level of one or more variables. Examples of variables used for biomarkers for particular diseases are systolic and diastolic blood pressure for hypertension, HbA1c level for type 2 diabetes, coronary calcium score for coronary artery disease, and high-sensitivity cardiac troponin T for acute myocardial infarction. The usefulness of such biomarkers in making a definitive finding about presence or absence of a disease or condition varies; many are used in conjunction with information from other tests or patient risk factors. Biomarkers used to detect diseases have distributions in non-diseased as well as in diseased populations. For most diseases, these distributions overlap, so that a single cutoff level does not clearly separate non-diseased from diseased people. For example, an HbA1c level of 6.5% may be designated as the cutoff point for diagnosing type 2 diabetes. In fact, some people whose HbA1c level is lower than 6.5% also have diabetes (as confirmed by other tests), and some people whose HbA1c level is higher than 6.5% do not have diabetes. Lowering the cutoff point to 6.0% or 5.5% will correctly identify more people who are diabetic, but it will also incorrectly identify more people as being diabetic who are not. For diabetes as well as other conditions, clinically useful cutoff points may vary among different population subgroups (e.g., by age or race/ethnicity).
A cutoff point that is set to detect more true positives will also yield more false positives; a cutoff point that is set to detect more true negatives will also yield more false negatives. There are various statistical approaches for determining “optimal” cutoff points, e.g., where the intent is to minimize total false positives and false negatives, with equal weight given to sensitivity and specificity (Perkins 2006). However, the selection of a cutoff point should consider the acceptable risks of false positives vs. false negatives. For example, if the penalty for a false negative test is high (e.g., in patients with a fatal disease for which there is an effective treatment), then the cutoff point is usually set to be highly sensitive to minimize false negatives. If the penalty for a false positive test is high (e.g., leading to confirmatory tests or treatments that are invasive, associated with adverse events, and expensive), then the cutoff point is usually set to be highly specific to minimize false positives. Given the different purposes of screening and diagnosis, and the associated penalties of false positives and false negatives, cutoff points may be set differently for screening and diagnosis of the same disease.
b. Tests and Health Outcomes
Beyond technical performance of screening and diagnostic tests, their effect on health outcomes or health-related quality of life is often less immediate or direct than for other types of technologies. The impacts of most preventive, therapeutic, and rehabilitative technologies on health outcomes can be assessed as direct cause-and-effect relationships between interventions and outcomes. However, the relationship between the use of screening and diagnostic tests and health outcomes is typically indirect, given intervening decisions or other steps between the test and health outcomes. Even highly accurate test results may be ignored or improperly interpreted by clinicians. Therapeutic decisions that are based on test results can have differential effects on patient outcomes. Also, the impact of those therapeutic decisions may be subject to other factors, such as patient adherence to a drug regimen. Even so, health care decision makers and policymakers increasingly seek direct or indirect evidence demonstrating that a test is likely to have an impact on clinical decisions and health care outcomes.
The effectiveness (or efficacy) of a diagnostic (or screening) technology can be determined along a chain of inquiry that leads from technical capacity of a technology to changes in patient health outcomes to cost effectiveness (where relevant to decision makers), as follows.
- Technical capacity. Does the technology perform reliably and deliver accurate information?
- Diagnostic accuracy. Does the technology contribute to making an accurate diagnosis?
- Diagnostic impact. Do the diagnostic results influence use of other diagnostic technologies, e.g., does it replace other diagnostic technologies?
- Therapeutic impact. Do the diagnostic findings influence the selection and delivery of treatment?
- Patient outcome. Does use of the diagnostic technology contribute to improved health of the patient?
- Cost effectiveness. Does use of the diagnostic technology improve the cost effectiveness of health care compared to alternative interventions?
If a diagnostic technology is not effective at any step along this chain, then it is not likely to be effective at any subsequent step. Effectiveness at a given step does not imply effectiveness at a later step (Feeny 1986; Fineberg 1977; Institute of Medicine 1985). An often-cited hierarchy of studies for assessing diagnostic imaging technologies that is consistent with the chain of inquiry noted above is shown in Box II-11. A generic analytical framework of the types of evidence questions that could be asked about the impacts of a screening test is presented in Box II-12. Some groups have developed standards for assessing the quality of studies of the accuracy of screening and diagnostic tests, such as for conducting systematic reviews of the literature on those tests (Smidt 2006; Whiting 2011).
Box II-11. Hierarchical Model of Efficacy for Diagnostic Imaging: Typical Measures of Analysis
Level 1. Technical efficacy
- Resolution of line pairs
- Modulation transfer function change
- Gray-scale range
- Amount of mottle
- Sharpness
Level 2. Diagnostic accuracy efficacy
- Yield of abnormal or normal diagnoses in a case series
- Diagnostic accuracy (% correct diagnoses in case series)
- Sensitivity and specificity in a defined clinical problem setting
- Measures of area under the ROC curve
Level 3. Diagnostic thinking efficacy
- Number (%) of cases in a series in which image judged "helpful" to making the diagnosis
- Entropy change in differential diagnosis probability distribution
- Difference in clinicians' subjectively estimated diagnosis probabilities pre- to post-test information
- Empirical subjective log-likelihood ratio for test positive and negative in a case series
Level 4. Therapeutic efficacy
- Number (%) of times image judged helpful in planning management of patient in a case series
- % of times medical procedure avoided due to image information
- Number (%) of times therapy planned before imaging changed after imaging information obtained (retrospectively inferred from clinical records)
- Number (%) of times clinicians' prospectively stated therapeutic choices changed after information obtained
Level 5. Patient outcome efficacy
- % of patients improved with test compared with/without test
- Morbidity (or procedures) avoided after having image information
- Change in quality-adjusted life expectancy
- Expected value of test information in quality-adjusted life years (QALYs)
- Cost per QALY saved with imaging information
- Patient utility assessment; e.g., Markov modeling; time trade-off
Level 6. Societal efficacy
- Benefit-cost analysis from societal viewpoint
- Cost-effectiveness analysis from societal viewpoint
Source: Thornbury JR, Fryback DG. Technology assessment − An American view. Eur J Radiol. 1992;14(2):147-56.
Box II-12. Example of Analytical Framework of Evidence Questions: Screening
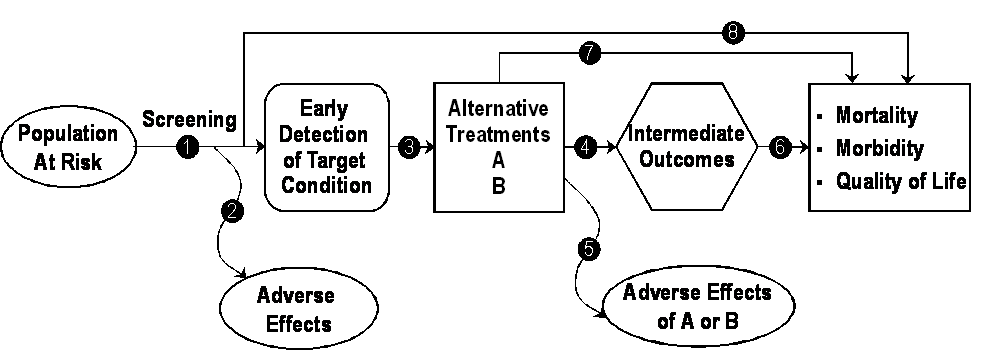
- Is screening test accurate for target condition?
- Does screening result in adverse effects?
- Do screening test results influence treatment decisions?
- Do treatments change intermediate outcomes?
- Do treatments result in adverse effects?
- Do changes in intermediate outcomes predict changes in health outcomes?
- Does treatment improve health outcomes?
- Is there direct evidence that screening improves health outcomes?
Source: Adapted from: Harris RP, Helfand M, Woolf SH, et al. Current methods of the US Preventive Services Task Force. A review of the process. Am J Prev Med. 2001;20(3S):21-35.
For diagnostic (or screening) technologies that are still prototypes or in other early stages of development, there may be limited data on which to base answers to such questions as these. Even so, investigators and advocates of diagnostic technologies should be prepared to describe, at least qualitatively, how the technology might affect diagnostic accuracy, diagnostic impact, therapeutic impact, patient outcomes and cost effectiveness (where appropriate); how these effects might be measured; approximately what levels of performance would be needed to successfully implement the technology; and how further investigations should be conducted to make these determinations.
3. Timing of Assessment
There is no single correct time to conduct an HTA. It is conducted to meet the needs of a variety of policymakers seeking assessment information throughout the lifecycles of technologies. Regulators, payers, clinicians, hospital managers, investors, and others tend to make decisions about technologies at particular junctures, and each may subsequently reassess technologies. Indeed, the determination of a technology's stage of diffusion may be the primary purpose of an assessment. For insurers and other payers, technologies that are deemed “experimental” or “investigational” are usually excluded from coverage, whereas those that are established or generally accepted are usually eligible for coverage (Newcomer 1990; Reiser 1994; Singer 2001).
There are tradeoffs inherent in decisions regarding the timing for HTA. On one hand, the earlier a technology is assessed, the more likely its diffusion can be curtailed if it is unsafe or ineffective (McKinlay 1981). From centuries’ old purging and bloodletting to the more recent autologous bone marrow transplantation with high-dose chemotherapy for advanced breast cancer, the list of poorly evaluated technologies that diffused into general practice before being found to be ineffective and/or harmful continues to grow. Box II-13 shows examples of health care technologies found to be ineffective or harmful after being widely diffused.
Box II-13. Technologies Found to be Ineffective or Harmful for Some or
All Indications After Diffusion
- Autologous bone marrow transplantation with high-dose chemotherapy for advanced breast cancer
- Antiarrhythmic drugs
- Bevacizumab for metastatic breast cancer
- Colectomy to treat epilepsy
- Diethylstilbestrol (DES) to improve pregnancy outcomes
- Electronic fetal monitoring during labor without access to fetal scalp sampling
- Episiotomy (routine or liberal) for birth
- Extracranial-intracranial bypass to reduce risk of ischemic stroke
- Gastric bubble for morbid obesity
- Gastric freezing for peptic ulcer disease
- Hormone replacement therapy for preventing heart disease in healthy menopausal women
- Hydralazine for chronic heart failure
- Intermittent positive pressure breathing
- Mammary artery ligation for coronary artery disease
- Magnetic resonance imaging (routine) for low back pain in first 6 weeks
- Optic nerve decompression surgery for nonarteritic anterior ischemic optic neuropathy
- Oxygen supplementation for premature infants
- Prefrontal lobotomy for mental disturbances
- Prostate-specific antigen (PSA) screening for prostate cancer
- Quinidine for suppressing recurrences of atrial fibrillation
- Radiation therapy for acne
- Rofecoxib (COX-2 inhibitor) for anti-inflammation
- Sleeping face down for healthy babies
- Supplemental oxygen for healthy premature babies
- Thalidomide for sedation in pregnant women
- Thymic irradiation in healthy children
- Triparanol (MER-29) for cholesterol reduction
Sources: Chou 2011; Coplen 1990; Enkin 2000; Feeny 1986; FDA Center for Drug Evaluation and Research 2010; Fletcher 2002; Grimes 1993; Mello 2001; The Ischemic Optic Neuropathy Decompression Trial Research Group 1995; Jüni 2004; Passamani 1991; Peters 2005; Rossouw 2002; Srinivas 2012; Toh 2010; US DHHS1990, 1993; others.
On the other hand, to regard the findings of an early assessment as definitive or final may be misleading. An investigational technology may not yet be perfected; its users may not yet be proficient; its costs may not yet have stabilized; it may not have been applied in enough circumstances to recognize its potential benefits; and its long-term outcomes may not yet be known (Mowatt 1997). As one technology assessor concluded about the problems of when-to-assess: “It’s always too early until, unfortunately, it’s suddenly too late!” (Buxton 1987). Further, the “moving target problem” can complicate HTA. By the time a HTA is conducted, reviewed, and disseminated, its findings may be outdated by changes in a technology, how it is used, its competing technologies (comparators) for a given health problem (indication), the health problems for which it is used, and other factors (Goodman 1996). See chapter VI, Determine Topics for HTA, for further discussion of identification of candidate assessment topics, horizon scanning, setting assessment priorities, reassessment, and the moving target problem.
In recent years, the demand for HTA by health care decision makers has increasingly involved requests for faster responses to help inform emergent decisions. This has led to development of “rapid HTAs” that are more focused, less-comprehensive assessments designed to provide high-level responses to such decision maker requests within approximately four-to-eight weeks. See discussion of rapid HTA in chapter X, Selected Issues in HTA.
Among the factors affecting the timing of HTA is the sufficiency of evidence to undertake an HTA. One of the types of circumstances in which there are tradeoffs in “when to assess” is a coverage decision for a new technology (or new application of an existing technology) for which there is promising, yet non-definitive or otherwise limited, evidence. For some of these technologies, delaying any reimbursement until sufficient evidence is available for a definitive coverage decision could deny access for certain patients with unmet medical need who might benefit. Further, the absence of any reimbursement could slow the generation of evidence. In such instances, payers may provide for coverage with evidence development or other forms of managed entry of the technology in which reimbursement is made for particular indications or other well-defined uses of the technology in exchange for collection of additional evidence. See further discussion of managed entry in chapter X.
D. Expertise for Conducting HTA
Given the variety of impacts addressed and the range of methods that may be used in an assessment, multiple types of experts are needed in HTA. Depending upon the topic and scope of assessment, these include a selection of the following:
- Physicians, nurses, other clinicians
- Managers of hospitals, clinics, nursing homes, and other health care institutions
- Pharmacists and pharmacologists
- Laboratory technicians, radiology technicians, and other allied health professionals
- Biomedical and clinical engineers
- Patients and community representatives
- Epidemiologists
- Biostatisticians
- Economists
- Social scientists
- Decision scientists
- Ethicists
- Lawyers
- Computer scientists/programmers
- Librarians/information specialists
Of course, certain individuals have multiple types of expertise. The set of participants in an HTA depends on the scope and depth of the topic, available resources, and other factors. For example, the standing members of a hospital technology assessment committee might include: the chief executive officer, chief financial officer, physician chief of staff, director of nursing, director of planning, materials manager, and director of biomedical engineering (Sadock 1997; Taylor 1994). Certain clinical specialists, and marketing, legal, and analytical staff and patient or community representatives could be involved as appropriate.
E. Basic HTA Frameworks
There is great variation in the scope, selection of methods and level of detail in the practice of HTA. Nevertheless, most HTA activity involves some form of the following basic steps.
1. Identify assessment topics
2. Specify the assessment problem or questions
3. Determine organizational locus or responsibility for assessment
4. Retrieve available relevant evidence
5. Generate or collect new evidence (as appropriate)
6. Appraise/interpret quality of the evidence
7. Integrate/synthesize evidence
8. Formulate findings and recommendations
9. Disseminate findings and recommendations
10. Monitor impact
Not all assessment programs conduct all of these steps, and they are not necessarily conducted in a linear manner. Many HTA programs rely largely on integrative methods of reviewing and synthesizing data (using systematic reviews and meta-analyses) based on existing relevant primary data studies (reported in journal articles or from epidemiological or administrative data sets). Some assessment efforts involve multiple cycles of retrieving/collecting, interpreting, and integrating evidence before completing an assessment. The steps of appraising and integrating evidence may be done iteratively, such as when individual primary data studies pertaining to a particular evidence question are appraised individually for quality and then are integrated into a body of evidence, which in turn is appraised for its overall quality, as described in chapter III and chapter IV. Depending on the circumstances of an HTA, the dissemination of findings and recommendations and monitoring of impact may not be parts of the HTA itself, although they may be important responsibilities of the sponsoring program or parent organization. As indicated by various chapter and section headings, all ten of the basic steps of HTA listed above are described in this document.
EUnetHTA has developed a “core model” for HTA to serve as a generic framework to enable international collaboration for producing and sharing the results of HTAs (EUnetHTA 2013). Core HTAs are intended to serve as a basis for local (i.e., a particular nation, region, or program) reports, and as such do not contain recommendations on technology use. The core model involves the following domains and production phases (EUnetHTA 2008; Lampe 2009):
EUnetHTA Core Model Domains
1. Health problem and current use of technology
2. Description and technical characteristics of technology
3. Safety
4. Clinical effectiveness
5. Costs and economic evaluation
6. Ethical analysis
7. Organizational aspects
8. Social aspects
9. Legal aspects
EUnetHTA Core Model Phases
1. Definition of the technology to be assessed
2. Definition of project type
3. Relevance of assessment elements
4. Translation of relevant issues into research questions
5. Compiling of a core HTA protocol
6. Research
7. Entering the results
HTA embraces a diverse group of methods. Two of the main types of HTA methods are primary data collection methods and secondary or integrative methods. Primary data methods (described in chapter III) involve collection of original data, such as clinical trials and observational studies. Integrative methods, or secondary or synthesis methods (chapter IV), involve combining data or information from existing sources, including from primary data studies. (Economic analysis methods, chapter V) can involve one or both of primary data methods and integrative methods.
Most HTA programs use integrative approaches, with particular attention to formulating findings that are based on distinguishing between stronger and weaker evidence drawn from available primary data studies. Some HTA programs do collect primary data, or are part of larger organizations that collect primary data. It is not always possible to conduct, or base an assessment on, the most rigorous types of studies. Indeed, policies often must be made in the absence, or before completion, of definitive studies. Given their varying assessment orientations, resource constraints and other factors, HTA programs tend to rely on different combinations of methods. Even so, the general trend in HTA is to call for and emphasize evidence based on the more rigorous and systematic methods.
References for Chapter II
Andersson I, Aspegren K, Janzon L, et al. Mammographic screening and mortality from breast cancer: The Malmö Mammographic Screening Trial. BMJ. 1988; 297(6654):943-8. PubMed | PMC free article.
Banta HD, Luce BR. Health Care Technology and Its Assessment: An International Perspective. New York, NY: Oxford University Press; 1993.
Bergner M, Bobbitt RA, Carter WB, Gilson BS. The Sickness Impact Profile: development and final revision of a health status measure. Med Care. 1981;19(8):787-805.
Biomarkers Definitions Working Group. Biomarkers and surrogate endpoints: preferred definitions and conceptual framework. Clin Pharmacol Ther. 2001;69(3):89-95. PubMed
Bleichrodt H, Johannesson M. Standard gamble, time trade-off and rating scale: experimental results on the ranking properties of QALYs. J Health Econ. 1997;16(2):155-75. PubMed
Breitkreutz I, Anderson KC. Thalidomide in multiple myeloma--clinical trials and aspects of drug metabolism and toxicity. Expert Opin Drug Metab Toxicol. 2008;4(7):973-85. PubMed
Brethauer SA, Aminian A, Romero-Talamás H, Batayyah E, et al. Can diabetes be surgically cured? Long-term metabolic effects of bariatric surgery in obese patients with type 2 diabetes mellitus. Ann Surg. 2013;258(4):628-36. PubMed | PMC free article
Buxton MJ. Problems in the economic appraisal of new health technology: the evaluation of heart transplants in the UK. In Drummond MF, ed. Economic Appraisal of Health Technology in the European Community. Oxford, England. Oxford Medical Publications, 1987.
Chou R, Croswell JM, Dana T, et al. Screening for Prostate Cancer: A Review of the Evidence for the U.S. Preventive Services Task Force. Ann Intern Med. 2011 Dec 6;155(11):762-71. PubMed | Publisher free article
Coleman RE, Briner WH, Siegel BA. Clinical PET scanning. A short-lived orphan. Int J Technol Assess Health Care. 1992;8(4):610-22. PubMed
Coons SJ, Rao S, Keininger DL, Hays RD. A comparative review of generic quality-of-life instruments. Pharmacoeconomics 2000;17(1):13-35. PubMed
Coplen SE, Antman EM, Berlin JA, Hewitt P, Chalmers TC. Efficacy and safety of quinidine therapy for maintenance of sinus rhythm after cardioversion. A meta-analysis of randomized control trials. Circulation. 1990;82(4):1106-16. PubMed
de Bruin AF, de Witte LP, Stevens F, Diederiks JP. Sickness Impact Profile: the state of the art of a generic functional status measure. Soc Sci Med. 1992;35(8):1003-14. PubMed
Doctor JN, Bleichrodt H, Lin HJ. Health utility bias: a systematic review and meta-analytic evaluation. Med Decis Making. 2010;30(1):58-67. PubMed
Dolan P. Utilitarianism and the measurement and aggregation of quality-adjusted life years. Health Care Anal. 2001;9(1):65-76. PubMed
Doll H.A, Black NA, Flood AB, McPherson K. Criterion validation of the Nottingham health profile: patient views of surgery for benign prostatic hypertrophy. Soc Sci Med. 1993;37(1):115‑22. PubMed
Duthie K, Bond K. Improving ethics analysis in health technology assessment. Int J Technol Assess Health Care 2011;27(1):64-70. PubMed
Enkin M, Nelison J, Crowther C, Duley L, et al. A Guide to Effective Care in Pregnancy and Childbirth. 3rd ed. New York, NY: Oxford University Press; 2000.
EUnetHTA (European Network for Health Technology Assessment). HTA Core Model for Medical and Surgical Interventions Version 1.0 Work Package 4. The HTA Core Model. December 2008. Publisher free publication
EUnetHTA. HTA Core Model® Online. National Institute for Health and Welfare. Helsinki; 2019. Accessed June 18, 2014 at: http://www.htacoremodel.info/.
FDA Center for Drug Evaluation and Research. Memorandum to the File: BLA 125085 Avastin (bevacizumab). Regulatory Decision to Withdraw Avastin (bevacizumab) Firstline Metastatic Breast Cancer Indication December 15, 2010. Accessed Sept. 1, 2011 at: //www.fda.gov/downloads/Drugs/DrugSafety/PostmarketDrugSafetyInformationforPatientsandProviders/UCM237171.pdf.
Feeny D, Guyatt G, Tugwell P, eds. Health care Technology: Effectiveness, Efficiency, and Public Policy. Montreal, Canada: Institute for Research on Public Policy; 1986.
Feeny D, Spritzer K, Hays RD, Liu H, et al. Agreement about identifying patients who change over time: cautionary results in cataract and heart failure patients. Med Decis Making. 2012;32(2):273-86. PubMed | PMC free article
Fineberg HV, Bauman R, Sosman M. Computerized cranial tomography: effect on diagnostic and therapeutic plans. JAMA. 1977;238(3):224-7. PubMed
Fletcher SW, Colditz GA. Failure of estrogen plus progestin therapy for prevention. JAMA. 2002;288(3):366-8. PubMed
Forrow L, Taylor WC, Arnold RM. Absolutely relative: how research results are summarized can affect treatment decisions. Am J Med. 1992;92(2):121-94. PubMed
Fox-Rushby JA, Hanson K. Calculating and presenting disability adjusted life years (DALYs) in cost-effectiveness analysis. Health Policy Plan. 2001;16(3):326-31. PubMed | PMC free article
Frosch DL, Kaplan RM, Ganiats TG, Groessl EJ, Sieber WJ, Weisman MH. Validity of self-administered quality of well-being scale in musculoskeletal disease. Arthritis Rheum. 2004;51(1):28-33. PubMed | Publisher free article
Fryback DG, Dunham NC, Palta M, Hanmer J, et al. US norms for six generic health-related quality-of-life indexes from the National Health Measurement study. Med Care. 2007;45(12):1162-70. PubMed | PMC free article
Fu TC, Westergaard RP, Lau B, Celentano DD, Vlahov D, Mehta SH, Kirk GD. Changes in sexual and drug-related risk behavior following antiretroviral therapy initiation among HIV-infected injection drug users. AIDS. 2012;26(18):2383-91. Accessed June 18, 2014 at: //www.ncbi.nlm.nih.gov/pmc/articles/PMC3678983. PubMed
Gelijns A, Rosenberg N. The dynamics of technological change in medicine. Health Aff (Millwood). 1994;13(3):28-46. PubMed | Publisher free article
George SM. Millions of missing girls: from fetal sexing to high technology sex selection in India. Prenat Diagn. 2006 Jul;26(7):604-9. PubMed
Gibson JL, Martin DK, Singer PA. Priority setting for new technologies in medicine: a transdisciplinary study. BMC Health Serv Res. 2002;2(1):14. PubMed | PMC free article
Glasziou P. What is EBM? Evid Based Med. 2011;16(5):129-30. PubMed
Gold MR, Stevenson D, Fryback DG. HALYS and QALYS and DALYS, oh my: similarities and differences in summary measures of population health. Annu Rev Public Health. 2002;23:115-34. PubMed
Goodman C. The moving target problem and other lessons from percutaneous transluminal coronary angioplasty. In: A Szczepura, Kankaanpää J. Assessment of Health Care Technologies: Case Studies, Key Concepts and Strategic Issues. New York, NY: John Wiley & Sons; 1996:29-65.
Goodman CS. Technology assessment in healthcare: a means for pursuing the goals of biomedical engineering. Med Biolog Eng Comput. 1993;31(1):HTA3-10. PubMed
Green ED, Guyer MS; National Human Genome Research Institute. Charting a course for genomic medicine from base pairs to bedside. Nature. 2011;470(7333):204-13. PubMed
Gregorian RS, Golden KA, Bahce A, Goodman C, et al. Antidepressant-induced sexual dysfunction. Ann Pharmacother. 2002;36(10):1577-89. PubMed
Grimes DA. Technology follies: the uncritical acceptance of medical innovation. JAMA. 1993;269(23):3030-3. PubMed
Guyatt G, Rennie D, Meade MO, Cook DJ. Users' Guides to the Medical Literature: A Manual for Evidence-Based Clinical Practice, 2nd Edition. New York: McGraw-Hill, 2008.
Hackam DG, Eikelboom JW. Antithrombotic treatment for peripheral arterial disease. Heart. 2007;93(3):303-8. PubMed | PMC free article
Harris RP, Helfand M, Woolf SH, et al. Current methods of the US Preventive Services Task Force. A review of the process. Am J Prev Med. 2001;20(3S):21-35. PubMed
Hayes JH, Barry MJ. Screening for prostate cancer with the prostate-specific antigen test: a review of current evidence. JAMA. 2014;311(11):1143-9. PubMed
Heitman E. Ethical issues in technology assessment. Conceptual categories and procedural considerations. Int J Technol Assess Health Care. 1998;14(3):544-66. PubMed
Hilborne LH, Leape LL, Kahan JP, Park RE, et al. Percutaneous Transluminal Coronary Angioplasty: A Literature Review of Ratings of Appropriateness and Necessity. Santa Monica, Ca: RAND; 1991. Publisher free publication
Hollis A, Ahmed Z. Preserving antibiotics, rationally. N Engl J Med. 2013;369(26):2474-6. PubMed | Publisher free article]
Hsueh IP, Lin JH, Jeng JS, Hsieh CL. Comparison of the psychometric characteristics of the functional independence measure, 5 item Barthel index, and 10 item Barthel index in patients with stroke. J Neurol Neurosurg Psychiatry. 2002;73(2):188-90. PubMed | PMC free article
Hudson KL. Genomics, health care, and society. N Engl J Med. 2011;365(11):1033-41. PubMed | Publisher free article
Huesch MD. One and done? Equality of opportunity and repeated access to scarce, indivisible medical resources. BMC Med Ethics. 2012;13:11. PubMed | PMC free article
Institute of Medicine. Assessing Medical Technologies. Washington, DC: National Academy Press; 1985. Publisher free book]
Institute of Medicine. Clinical Practice Guidelines We Can Trust. Washington, DC: National Academies Press; 2011. Publisher free book
The Ischemic Optic Neuropathy Decompression Trial Research Group. Optic nerve decompression surgery for nonarteritic anterior ischemic optic neuropathy (NAION) is not effective and may be harmful. JAMA. 1995;273(8):625-32. PubMed
Jenkinson C, Fitzpatrick R, Argyle M. The Nottingham Health Profile: an analysis of its sensitivity to differentiating illness groups. Soc Sci Med. 1988;27(12):1411-4. PubMed
Johannesson M, Pliskin JS, Weinstein MC. Are healthy-years equivalents an improvement over quality-adjusted life years? Med Dec Making. 1993;13(4):281-6.PubMed
Jüni P, Nartey L, Reichenbach S, et al. Risk of cardiovascular events and rofecoxib: cumulative meta-analysis. Lancet. 2004;364(9450):2021-9. PubMed
Juniper EF, Guyatt GH, Ferrie PJ, Griffith LE. Measuring quality of life in asthma. Am Rev Respir Dis. 1993;147(4):832-8. PubMed
Juniper EF, Svensson K, Mörk AC, Ståhl E. Modification of the asthma quality of life questionnaire (standardised) for patients 12 years and older. Health Qual Life Outcomes. 2005;3:58. Pubmed | PMC free article.
Kahan JP, Bernstein SJ, Leape LL, Hilborne LH, Park RE, Parker L, Kamberg CJ, Brook RH. Measuring the necessity of medical procedures. Med Care. 1994;32(4):357-65. PubMed
Kaplan RM, Anderson JP, Wu AW, Mathews WC, Kozin F, Orenstein D. The Quality of Well-being Scale: applications in AIDS, cystic fibrosis, and arthritis. Med Care. 1989; 27(3 Suppl): S27-43. PubMed
Kaplan RM, Ganiats TG, Sieber WJ, Anderson JP. The Quality of Well-Being Scale: critical similarities and differences with SF-36. Int J Qual Health Care. 1998;10(6):509-20. PubMed | Publisher free article
Kaplan RM, Tally S, Hays RD, Feeny D, Ganiats TG, et al. Five preference-based indexes in cataract and heart failure patients were not equally responsive to change. J Clin Epidemiol 2011;64(5):497-506. PubMed | PMC free article
Kembabazi A, Bajunirwe F, Hunt PW, Martin JN, et al. Disinhibition in risky sexual behavior in men, but not women, during four years of antiretroviral therapy in rural, southwestern Uganda. PLoS One. 2013;8(7):e69634. PubMed | PMC free article
Kling J. From hypertension to angina to Viagra. Mod. Drug Discov. 1998;1(2):31-8.
Kuperman GJ, Blair JS, Franck RA, Devaraj S, Low AF; NHIN Trial Implementations Core Services Content Working Group. Developing data content specifications for the nationwide health information network trial implementations. J Am Med Inform Assoc. 2010;17(1):6-12. PubMed | PMC free article
The Lewin Group. Outlook for Medical Technology Innovation. Report 4: The Impact of Regulation and Market Dynamics on Innovation. Washington, DC: AdvaMed; 2001.
Lalezari JP, Henry K, O’Hearn M, et al. Enfuvirtide, an HIV-1 fusion inhibitor, for drug-resistant HIV infection in North and South America. N Engl J Med. 2003;348(22):2175-85. PubMed | Publisher free article
Lampe K, Mäkelä M, Garrido MV, et al.; European network for Health Technology Assessment (EUnetHTA). The HTA core model: a novel method for producing and reporting health technology assessments. Int J Technol Assess Health Care. 2009;25 Suppl 2:9-20. PubMed
Lauritsen KJ, Nguyen T. Combination products regulation at the FDA. Clin Pharmacol Ther. 2009;85(5):468-70. PubMed
Lechler RI, Sykes M, Thomson AW, Turka LA. Organ transplantation--how much of the promise has been realized? Nat Med. 2005;11(6):605-13. PubMed
Lin K, Lipsitz R, Miller T, Janakiraman S; U.S. Preventive Services Task Force. Benefits and harms of prostate-specific antigen screening for prostate cancer: an evidence update for the U.S. Preventive Services Task Force. Ann Intern Med. 2008;149(3):192-9. PubMed
Linacre JM, Heinemann AW, Wright BD, Granger CV, Hamilton BB. The structure and stability of the Functional Independence Measure. Arch Phys Med Rehab. 1994;75(2):127-32. PubMed
Malyszko J, Muntner P, Rysz J, Banach M. Blood pressure levels and stroke: J-curve phenomenon? Curr Hypertens Rep. 2013;15(6):575-81. PubMed | PMC free article
Martin ML, Patrick DL, Gandra SR, Bennett AV, et al. Content validation of two SF-36 subscales for use in type 2 diabetes and non-dialysis chronic kidney disease-related anemia. Qual Life Res 2011;20(6):889-901. PubMed
Massa T. An industry perspective: challenges in the development and regulation of drug-device combination products. In Hanna K, Manning FJ, Bouxsein P, Pope A, eds. Innovation and Invention in Medical Devices. Workshop Summary. Institute of Medicine. Washington, DC: National Academy Press; 2001:16-20. Publisher free book
McKinlay JB. From “promising report” to “standard procedure”: seven stages in the career of a medical innovation. Milbank Memorial Fund Quarterly. 1981;59(3):374-411. PubMed
Meenan RF, Mason JH, Anderson JJ, Guccione AA, Kazis LE. AIMS2. The content and properties of revised and expanded arthritis impact measurement scales health status questionnaire. Arthritis Rheum. 1992;35(1):1-10. PubMed
Mello MM, Brennan TA. The controversy over high-dose chemotherapy with autologous bone marrow transplant for breast cancer. Health Aff (Millwood). 2001;20(5):101-17. PubMed | Publisher free article
Moss AH. Ethical principles and processes guiding dialysis decision-making. Clin J Am Soc Nephrol. 2011;6(9):2313-7. PubMed] | Publisher free article
Mowatt G, Bower DJ, Brebner JA, Cairns JA, et al. When and how to assess fast-changing technologies: a comparative study of medical applications of four generic technologies. Health Technol Assess. 1997;1(14). PubMed | Publisher free article
Mullahy J. Live long, live well: quantifying the health of heterogeneous populations. Health Econ. 2001;10(5):429-40. PubMed
Newcomer LN. Defining experimental therapy − a third party payer's dilemma. N Engl J Med. 1990;323(24):1702-4. PubMed
Nie JB. Non-medical sex-selective abortion in China: ethical and public policy issues in the context of 40 million missing females. Br Med Bull. 2011;98:7-20. PubMed | Publisher free article
Nord E, Enge AU, Gundersen V. QALYs: is the value of treatment proportional to the size of the health gain? Health Econ. 2010;19(5):596-607. PubMed
Oldridge N, Furlong W, Perkins A, Feeny D, Torrance GW. Community or patient preferences for cost-effectiveness of cardiac rehabilitation: does it matter? Eur J Cardiovasc Prev Rehabil. 2008;15(5):608-15. PubMed
Passamani E. Clinical trials: are they ethical? N Engl J Med. 1991;324(22):1589-92. PubMed
Patrick DL, Martin ML, Bushnell DM, Marquis P, Andrejasich CM, Buesching DP: Cultural adaptation of a quality-of-life measure for urinary incontinence. Eur Urol. 1999;36(5):427-35. PubMed
Patrick DL, Starks HE, Cain KC, Uhlmann RF, Pearlman RA. Measuring preferences for health states worse than death. Med Dec Making. 1994;14(1):9-18. PubMed
Perkins NJ, Schisterman EF. The inconsistency of "optimal" cutpoints obtained using two criteria based on the receiver operating characteristic curve. Am J Epidemiol. 2006;163(7):670-5. PubMed | PMC free article
Peters WP, Rosner GL, Vredenburgh JJ, et al. Prospective, randomized comparison of high-dose chemotherapy with stem-cell support versus intermediate-dose chemotherapy after surgery and adjuvant chemotherapy in women with high-risk primary breast cancer: a report of CALGB 9082, SWOG 9114, and NCIC MA-13. J Clin Oncol. 2005;23(10):2191-200. PubMed
Post MW, Gerritsen J, Diederikst JP, DeWittet LP. Measuring health status of people who are wheelchair-dependent: validity of the Sickness Impact Profile 68 and the Nottingham Health Profile. Disabil Rehabil. 2001;23(6):245-53. PubMed
Potter BK, Avard D, Graham ID, et al. Guidance for considering ethical, legal, and social issues in health technology assessment: application to genetic screening. Int J Technol Assess Health Care. 2008;24(4):412-22. PubMed
Rabin R, de Charro F. EQ-5D: a measure of health status from the EuroQol Group. Ann Med. 2001;33(5):337-43. PubMed
Reiser SJ. Criteria for standard versus experimental therapy. Health Aff (Millwood). 1994;13(3):127-36. PubMed Publisher free article
Rettig RA, Jacobson PD, Farquhar CM, Aubry WM. False Hope: Bone Marrow Transplantation for Breast Cancer. New York: Oxford University Press; 2007.
Rettig RA, Levinsky NG, eds. Kidney Failure and the Federal Government. Washington, DC: National Academy Press; 1991. PubMed | Publisher free book
Reuzel RP, van der Wilt GJ, ten Have HA, de Vries Robbe PF. Interactive technology assessment and wide reflective equilibrium. J Med Philos. 2001;26(3):245-61. PubMed
Rossouw JE, Anderson GL, Prentice RL, et al. Risks and benefits of estrogen plus progestin in healthy postmenopausal women: principal results from the Women's Health Initiative randomized controlled trial. JAMA. 2002;288(3):321-33. PubMed
Saarni SI, Braunack-Mayer A, Hofmann B, van der Wilt GJ. Different methods for ethical analysis in health technology assessment: An empirical study. Int J Technol Assess Health Care 2011;27(4):305-12. PubMed
Saban KL, Stroupe KT, Bryant FB, Reda DJ, et al. Comparison of health-related quality of life measures for chronic renal failure: quality of well-being scale, short-form-6D, and the kidney disease quality of life instrument. Qual Life Res. 2008;17(8):1103-15.PubMed
Sackett DL, Richardson WS, Rosenberg W, Haynes RB. Evidence-Based Medicine. New York, NY: Churchill Livingstone, 1997.
Sadock J, Tolman ED. Capital equipment budgeting: changing an art to a science. J Healthc Resour Manag. 1997;15(8):16-21. PubMed
Sassi F. Setting priorities for the evaluation of health interventions: when theory does not meet practice. Health Policy. 2003;63(2):141-54. PubMed
Schwappach DL. Resource allocation, social values and the QALY: a review of the debate and empirical evidence. Health Expect. 2002;5(3):210-22. PubMed
Singer SJ, Bergthold LA. Prospects for improved decision making about medical necessity. Health Aff (Millwood). 2001;20(1):200-6. PubMed | Publisher free article
Smidt N, Rutjes AW, van der Windt DA, Ostelo RW, et al. Reproducibility of the STARD checklist: an instrument to assess the quality of reporting of diagnostic accuracy studies. BMC Med Res Methodol. 2006;6:12. PubMed | PMC free article
Söderlin MK, Kautiainen H, Skogh T, Leirisalo-Repo M. Quality of life and economic burden of illness in very early arthritis. A population based study in southern Sweden. J Rheumatol 2004;31(9):1717-22. PubMed
Soto-Gutierrez A, Wertheim JA, Ott HC, Gilbert TW. Perspectives on whole-organ assembly: moving toward transplantation on demand. J Clin Invest. 2012;122(11):3817-23. PubMed | PMC free article
Sox H, Stern S, Owens D, Abrams HL. Assessment of Diagnostic Technology in Health Care: Rationale, Methods, Problems, and Directions. Institute of Medicine. Washington, DC: National Academy Press; 1989.
Srinivas SV, Deyo RA, Berger ZD. Application of "less is more" to low back pain. Arch Intern Med. 2012;172(13):1016-20. PubMed
Stamuli E. Health outcomes in economic evaluation: who should value health? Br Med Bull. 2011;97:197-210. PubMed
Straus SE, Richardson WS, Glasziou P, Haynes RB. Evidence-Based Medicine: How to Practice and Teach It. 4th ed. New York, NY: Churchill Livingstone Elsevier, 2011.
Taylor KS. Hospital tech assessment teams field requests − and politics. Hosp Health Netw. 1994;68(16):58. PubMed
Thiele AT, Leier B. Towards an ethical policy for the prevention of fetal sex selection in Canada. J Obstet Gynaecol Can. 2010 Jan;32(1):54-7. PubMed
Thornbury JR, Fryback DG. Technology assessment − An American view. Eur J Radiol. 1992;14(2):147-56. PubMed
Toh S, Hernández-Díaz S, Logan R, Rossouw JE, Hernán MA. Coronary heart disease in postmenopausal recipients of estrogen plus progestin therapy: does the increased risk ever disappear? A randomized trial. Ann Intern Med. 2010;152(4):211-7. PubMed | PMC free article
Torrance GW, Feeny D. Utilities and quality-adjusted life years. Int J Technol Assess Health Care. 1989;5(4):559-75. PubMed
Tun W, Gange SJ, Vlahov D, Strathdee SA, Celentano DD. Increase in sexual risk behavior associated with immunologic response to highly active antiretroviral therapy among HIV-infected injection drug users. Clin Infect Dis. 2004;38(8):1167-74. PubMed | Publisher free article
US Congress, House of Representatives. Committee on Science and Astronautics. Technology Assessment. Statement of Emilio Q. Daddario, Chairman, Subcommittee on Science Research and Development. 90th Cong., 1st sess., Washington, DC; 1967.
US Department of Health and Human Services, Agency for Health Care Policy and Research. Extracranial-Intracranial Bypass to Reduce the Risk of Ischemic Stroke. Health Technology Assessment Reports. No. 6. Rockville, Md; 1990. Bookshelf free publication
US Department of Health and Human Services, Agency for Health Care Policy and Research. Intermittent Positive Pressure Breathing: Old Technologies Rarely Die. Rockville, MD; 1993. Publisher free publication
US Food and Drug Administration. Guidance for Industry and FDA Staff. Pharmacogenetic Tests and Genetic Tests for Heritable Markers. June 19, 2007. Accessed June 18, 2014 at: //www.fda.gov/downloads/MedicalDevices/DeviceRegulationandGuidance/GuidanceDocuments/ucm071075.pdf
Van der Wilt GJ, Reuzel R, Banta HD. The ethics of assessing health technologies. Theor Med Bioeth. 2000;21(1):103-15. PubMed
Wagner TH, Patrick DL, Bavendam TG, Martin ML, Buesching DP: Quality of life in persons with urinary incontinence: development of a new measure. Urology 1996;47(1):67-72. PubMed
Ware JE Jr., Sherbourne CD. The MOS 36-item Short Form Health Survey (SF-36): I. conceptual framework and item selection. Med Care. 1992;30(6):473‑83. PubMed
Weinstein MC, Skinner JA. Comparative effectiveness and health care spending--implications for reform. N Engl J Med. 2010;362(5):460-5. PubMed | PMC free article
Whitehead SJ, Ali S. Health outcomes in economic evaluation: the QALY and utilities. Br Med Bull 2010;96:5-21. PubMed | Publisher free article
Whiting PF, Rutjes AW, Westwood ME, et al.; QUADAS-2 Group. QUADAS-2: a revised tool for the quality assessment of diagnostic accuracy studies. Ann Intern Med. 2011;155(8):529-36. PubMed
Yang H. Let genomics go global. The World in 2013. Economist. Nov. 21, 2012. Accessed November 18, 2014 at: http://www.economist.com/news/21566443-life-sciences-are-ready-revolution-it-will-require-collaboration-many-fronts-says-yang.
Yoshida EM. Selecting candidates for liver transplantation: a medical ethics perspective on the microallocation of a scarce and rationed resource. Can J Gastroenterol. 1998;12(3):209-15. PubMed
Zhou S, Wang F, Hsieh TC, Wu JM, Wu E. Thalidomide-a notorious sedative to a wonder anticancer drug. Curr Med Chem. 2013;20(33):4102-8. PubMed | PMC free article
Page 1 of 1
Last Reviewed: November 21, 2019